Enterprise
Tapping into Hidden Knowledge: Building an AI-Powered Knowledge Base for Your Enterprise
Dec 19, 2024
5 min read
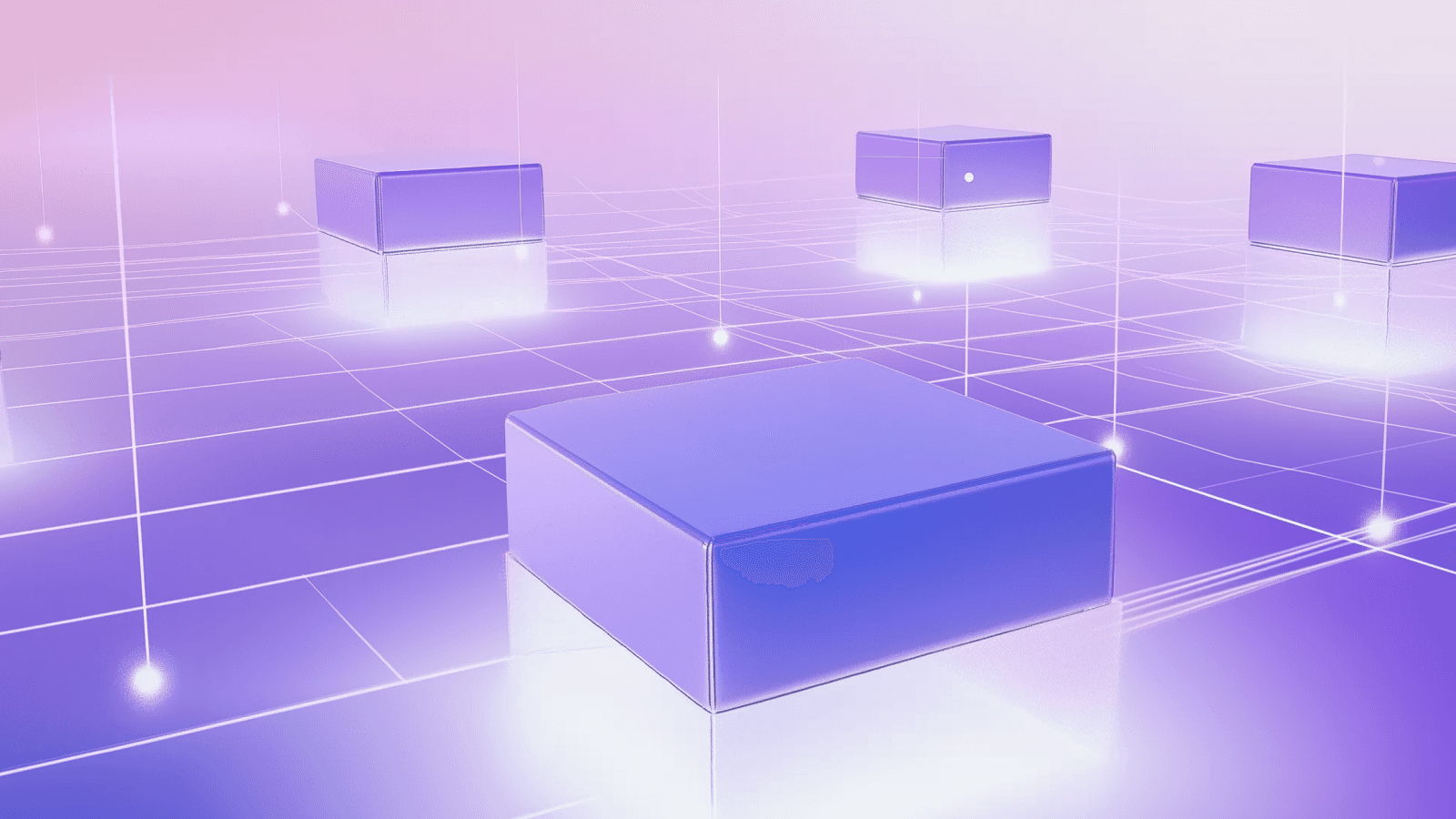
Every enterprise’s harsh reality is that they face immense competition every day. There are 520 payment software companies, 466 project management tools, 4012 commercial banks and 33 million small businesses in the US.
Whether you’re making toothpaste or building software, competition is inevitable. As a result, the differentiation in products or features is minimal. Your biggest differentiator - and competitive advantage - is the intangibles, like customer experience, ease of use, personalization, etc.
In short, your success depends not on what you do, but ‘how’ you do things.
But, in an organization of thousands of people, how do you ensure that everyone practices the same culture, embodies the same values, and speaks the same language?
The simple answer is creating common institutional knowledge, where everyone knows what they need to know. That’s easier said than done.
Codifying your how: Traditional learning initiatives
Traditionally, institutional knowledge was imparted through operating manuals, seminars, onboarding lectures, and on-the-job training. The challenge with such outbound knowledge transfer is:
Only the known-knowns are covered
Information given is often generic and not contextual
People forget most of what they learned the moment they step out of the class
And then, the gap between knowing something and applying it to practice is huge. The few examples and workshopping done during training sessions don’t cover every workplace scenario.
For instance, your new employee might learn in training that transparency is a key value in your organization. But what does that mean? Do you share passwords transparently? Do you publish salary data? Do you discuss politics? Do you speak your mind and offer feedback about work?
In response to these challenges, the traditional knowledge base emerged. Originating in the world of computer science — now used widely — a knowledge base is a hierarchical and structured way to store information. A typical knowledge base was like an employee handbook.
The good news is that traditional knowledge bases made information available to the user when they want it. They were indexed and searchable. As the knowledge economy grew, this became common practice.
Yet challenges remain:
Still restricted to known-knowns
Used only structured and documented information
Looking for information was time-consuming
And the employee was entirely alone in their search for knowledge. Unless they asked someone who was kind enough to help, a lot of information was lost in translation. Worse still, for aspects that aren’t deliberately codified, employees saw and learned.
Such unconscious mimicking of behavior has its troubles. Without deliberation and validation, employees might pick up on things that weren’t meant to be, creating counterproductive knowledge.
As author and consultant Ron Ashkenas, writes in the Harvard Business Review, while some institutional knowledge “gets translated into procedures and policies, most of it resides in the heads, hands, and hearts of individual managers and functional experts." Over the years, this knowledge moves away, degrades, or entirely disappears in the natural course of business.
A giant leap forward with an AI knowledge base
Obviously, AI isn’t the bandaid to every problem. However, knowledge management is something AI can meaningfully help with. Here’s how.
Why do you need an AI knowledge base?
A traditional knowledge base is like a book you read to learn something. You can highlight, bookmark, add notes, and carry it in your bag, but that’s that. If you want to find something, you need to remember where, what, and how it was.
An AI knowledge base is like that wise senior employee who has the answers to all questions. They help solve problems and get things done (not just flash information). Often, they might even present answers you didn’t know to ask questions about.
In the real world, the differences are telling.
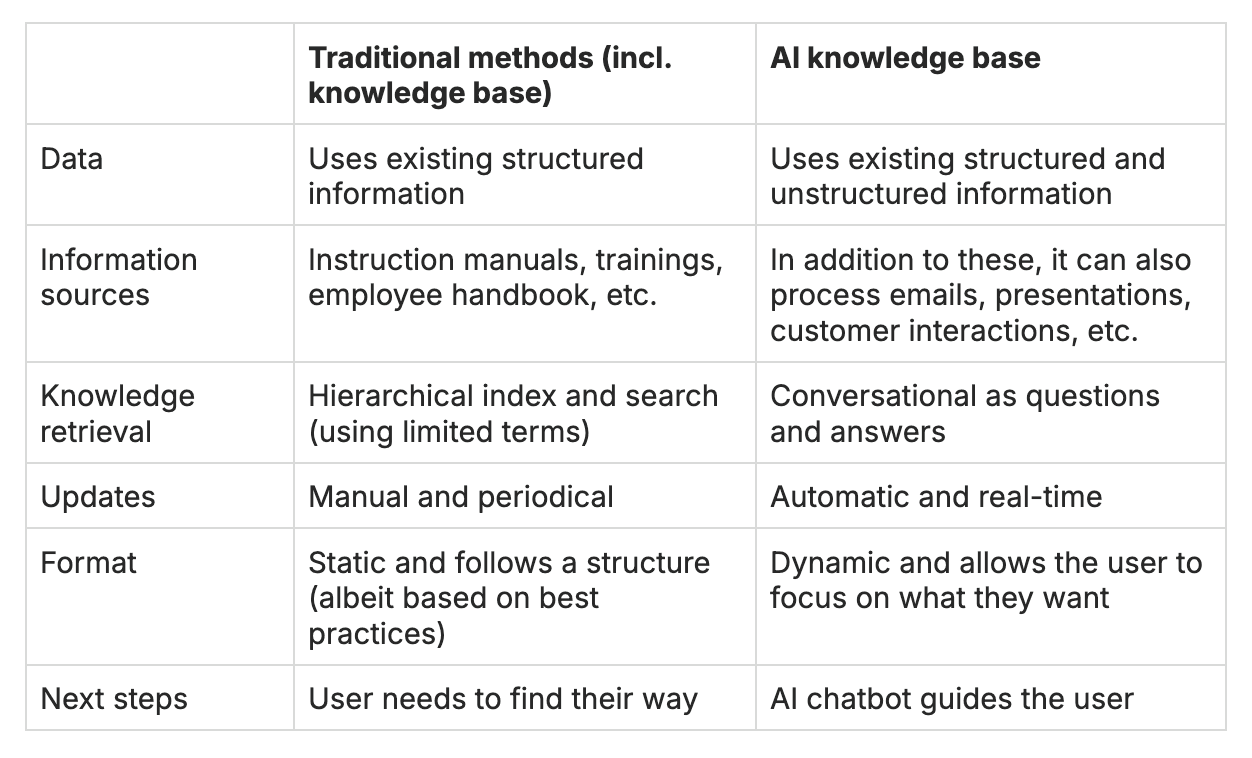
Who is an AI knowledge base for?
The users for an AI knowledge base can be anyone within and outside the organization, depending on your goals and needs. Let’s see some examples.
Customer: Interactions about product information, returns policies, pricing, subscriptions, discounts, etc.
Employee: Understanding company culture, behavior, policies, processes, etc. Imagine the reduction in HR workload if no one ever asked them any questions!
Vendors: Questions about contract renewal, payment timelines, updates, etc. Free up the procurement team of telling a 100 vendors each time that you pay on the first Thursday of every month.
Teams: Standups, updates, schedules, briefs, etc. For instance, integrating AI into your project management system can automate standup notes, understand each other’s work progress, etc. without intrusive conversations or huddles.
Organization: Random bits of information will continue to strengthen institutional knowledge, compounding over time.
How to create an AI knowledge base?
Enterprises already have comprehensive, structured knowledge. You most likely do, too. The best approach is to build on that. Here’s a strategy we’ve found success in implementing.
Define your goals
Ask yourself:
Who is it for? Existing employees, new employees, just the finance team, etc.
What should it do? Reduce emails to HR, save training budget, etc.
What questions should it answer? Boundaries of the knowledge this AI application will possess (critical for security, privacy and compliance)
Centralize your data
When you know the scope of your knowledge base, collect all the data you need for it. We’ve found that a majority of the information is in the following places:
HR platforms: The HRMS, payroll, performance management tools, etc., typically have data around the employee experience.
CX platforms: Customer support tools will have a comprehensive record of customer interactions across channels, including support history, resolutions offered, CSAT/NPS, etc.
Enterprise platforms: ERP, CRM, vendor management, compliance management, finance, etc.
Project management: Whether it’s custom tools like Jira or Trello or the good old Spreadsheet, project management (and internal communication) tools are a goldmine of information about ‘how’ you do things.
Documentation: Past proposals, pitches, presentations, contracts, etc. are stored in SharePoint, OneDrive, or Google Drive. Some of these might be text files, and others might be images, PDFs, etc.
Here’s how we built an agentic assistant for the sales team, leveraging knowledge from 10 years of proposals for a UAE-based event management company.
While doing this, think about access control and data ownership. Define who owns the data you’re integrating and who can access what parts of it. For instance, you might integrate the CRM, while not including any personally identifiable information to your AI solution.
Implement an agentic solution
You can choose something off-the-shelf and integrate all your data into it. However, over the long term, it helps to have a custom solution designed for you and implemented on your infrastructure.
User interface: Build the admin panel and the agentic assistant to enable your teams to use the AI knowledge base.
Design the AI solution: Set up the core servers, retrieval-augmented generation (RAG), feedback storage, database, etc. Choose the LLM and fine-tune it as needed.
Data sources: Integrate the relevant data sources and voila!
Set up governance
With great AI comes great compliance, security and governance risks. Make sure you have comprehensive preventative measures for your AI knowledge base. Some things to think about are:
Data access protocols
SSL/TLS
Validation, authentication and authorization
Encryption
Backups, retention, and geo-redundant storage
Secure cloud environments
Least privilege principle
Compliance requirements for GDPR, HIPAA, etc.
Enable continuous improvement
No AI solution is a one-and-done thing. In fact, good AI evolves and gets better with time. So, set up the protocols and processes for continuous improvement.
Get subject matter experts to perform random tests at regular intervals
Monitor continuously to gauge the performance of your knowledge base
Collect regular feedback on the accuracy, usability and usefulness of the AI knowledge base
Integrate new sources of data as and when they become available
Measure progress against business goals
It’s easy to think of AI knowledge bases as expenses without ROI. Don’t make that mistake. Review its performance on a regular basis. For instance, have the number of queries to the HR team reduced and by how much? Has the knowledge base helped reduce the training budget without affecting employee performance?
Use these metrics to troubleshoot and optimize your AI knowledge base.
For the enterprise team, this might be a lot, given the tech landscape they already manage. An AI knowledge base has a lot of moving parts that need to be balanced delicately. Tune AI’s experts are adept at exactly that.
If you’re looking to implement an AI knowledge base in your enterprise, speak to us today. We’ll show you how you can get it done faster, smarter and better.
Every enterprise’s harsh reality is that they face immense competition every day. There are 520 payment software companies, 466 project management tools, 4012 commercial banks and 33 million small businesses in the US.
Whether you’re making toothpaste or building software, competition is inevitable. As a result, the differentiation in products or features is minimal. Your biggest differentiator - and competitive advantage - is the intangibles, like customer experience, ease of use, personalization, etc.
In short, your success depends not on what you do, but ‘how’ you do things.
But, in an organization of thousands of people, how do you ensure that everyone practices the same culture, embodies the same values, and speaks the same language?
The simple answer is creating common institutional knowledge, where everyone knows what they need to know. That’s easier said than done.
Codifying your how: Traditional learning initiatives
Traditionally, institutional knowledge was imparted through operating manuals, seminars, onboarding lectures, and on-the-job training. The challenge with such outbound knowledge transfer is:
Only the known-knowns are covered
Information given is often generic and not contextual
People forget most of what they learned the moment they step out of the class
And then, the gap between knowing something and applying it to practice is huge. The few examples and workshopping done during training sessions don’t cover every workplace scenario.
For instance, your new employee might learn in training that transparency is a key value in your organization. But what does that mean? Do you share passwords transparently? Do you publish salary data? Do you discuss politics? Do you speak your mind and offer feedback about work?
In response to these challenges, the traditional knowledge base emerged. Originating in the world of computer science — now used widely — a knowledge base is a hierarchical and structured way to store information. A typical knowledge base was like an employee handbook.
The good news is that traditional knowledge bases made information available to the user when they want it. They were indexed and searchable. As the knowledge economy grew, this became common practice.
Yet challenges remain:
Still restricted to known-knowns
Used only structured and documented information
Looking for information was time-consuming
And the employee was entirely alone in their search for knowledge. Unless they asked someone who was kind enough to help, a lot of information was lost in translation. Worse still, for aspects that aren’t deliberately codified, employees saw and learned.
Such unconscious mimicking of behavior has its troubles. Without deliberation and validation, employees might pick up on things that weren’t meant to be, creating counterproductive knowledge.
As author and consultant Ron Ashkenas, writes in the Harvard Business Review, while some institutional knowledge “gets translated into procedures and policies, most of it resides in the heads, hands, and hearts of individual managers and functional experts." Over the years, this knowledge moves away, degrades, or entirely disappears in the natural course of business.
A giant leap forward with an AI knowledge base
Obviously, AI isn’t the bandaid to every problem. However, knowledge management is something AI can meaningfully help with. Here’s how.
Why do you need an AI knowledge base?
A traditional knowledge base is like a book you read to learn something. You can highlight, bookmark, add notes, and carry it in your bag, but that’s that. If you want to find something, you need to remember where, what, and how it was.
An AI knowledge base is like that wise senior employee who has the answers to all questions. They help solve problems and get things done (not just flash information). Often, they might even present answers you didn’t know to ask questions about.
In the real world, the differences are telling.
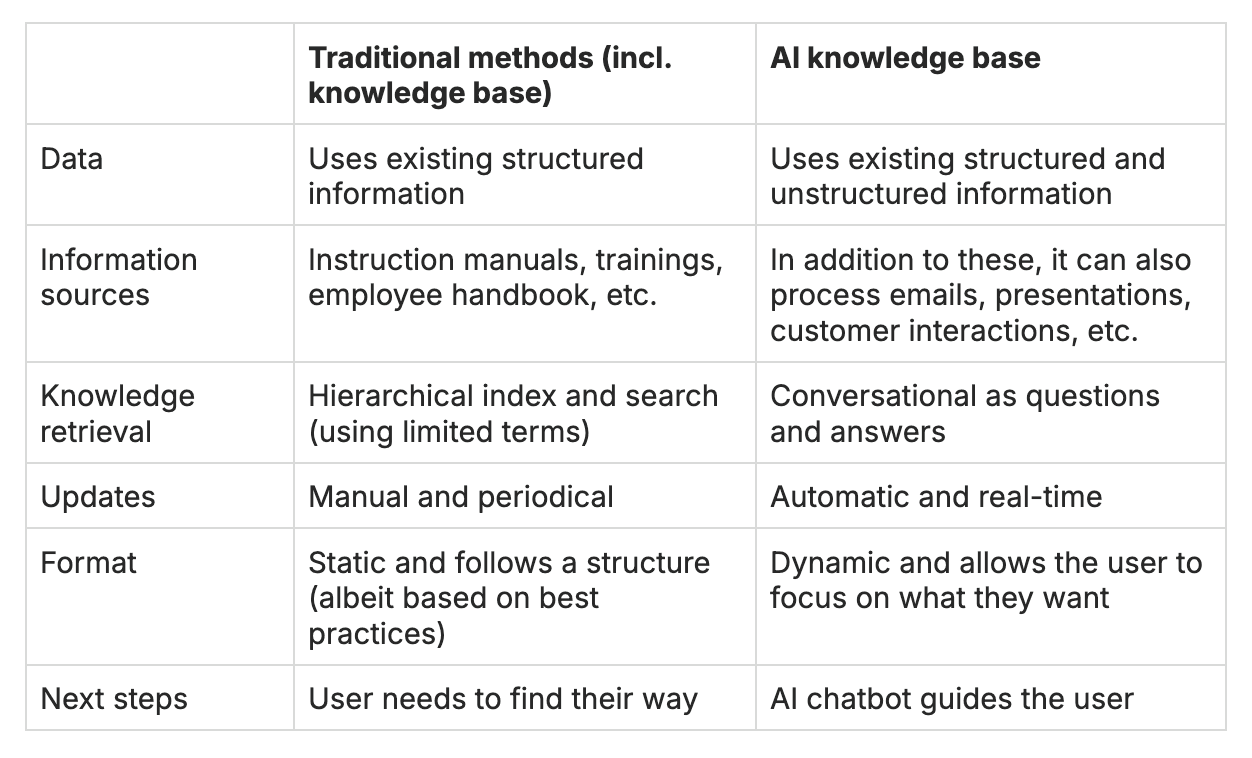
Who is an AI knowledge base for?
The users for an AI knowledge base can be anyone within and outside the organization, depending on your goals and needs. Let’s see some examples.
Customer: Interactions about product information, returns policies, pricing, subscriptions, discounts, etc.
Employee: Understanding company culture, behavior, policies, processes, etc. Imagine the reduction in HR workload if no one ever asked them any questions!
Vendors: Questions about contract renewal, payment timelines, updates, etc. Free up the procurement team of telling a 100 vendors each time that you pay on the first Thursday of every month.
Teams: Standups, updates, schedules, briefs, etc. For instance, integrating AI into your project management system can automate standup notes, understand each other’s work progress, etc. without intrusive conversations or huddles.
Organization: Random bits of information will continue to strengthen institutional knowledge, compounding over time.
How to create an AI knowledge base?
Enterprises already have comprehensive, structured knowledge. You most likely do, too. The best approach is to build on that. Here’s a strategy we’ve found success in implementing.
Define your goals
Ask yourself:
Who is it for? Existing employees, new employees, just the finance team, etc.
What should it do? Reduce emails to HR, save training budget, etc.
What questions should it answer? Boundaries of the knowledge this AI application will possess (critical for security, privacy and compliance)
Centralize your data
When you know the scope of your knowledge base, collect all the data you need for it. We’ve found that a majority of the information is in the following places:
HR platforms: The HRMS, payroll, performance management tools, etc., typically have data around the employee experience.
CX platforms: Customer support tools will have a comprehensive record of customer interactions across channels, including support history, resolutions offered, CSAT/NPS, etc.
Enterprise platforms: ERP, CRM, vendor management, compliance management, finance, etc.
Project management: Whether it’s custom tools like Jira or Trello or the good old Spreadsheet, project management (and internal communication) tools are a goldmine of information about ‘how’ you do things.
Documentation: Past proposals, pitches, presentations, contracts, etc. are stored in SharePoint, OneDrive, or Google Drive. Some of these might be text files, and others might be images, PDFs, etc.
Here’s how we built an agentic assistant for the sales team, leveraging knowledge from 10 years of proposals for a UAE-based event management company.
While doing this, think about access control and data ownership. Define who owns the data you’re integrating and who can access what parts of it. For instance, you might integrate the CRM, while not including any personally identifiable information to your AI solution.
Implement an agentic solution
You can choose something off-the-shelf and integrate all your data into it. However, over the long term, it helps to have a custom solution designed for you and implemented on your infrastructure.
User interface: Build the admin panel and the agentic assistant to enable your teams to use the AI knowledge base.
Design the AI solution: Set up the core servers, retrieval-augmented generation (RAG), feedback storage, database, etc. Choose the LLM and fine-tune it as needed.
Data sources: Integrate the relevant data sources and voila!
Set up governance
With great AI comes great compliance, security and governance risks. Make sure you have comprehensive preventative measures for your AI knowledge base. Some things to think about are:
Data access protocols
SSL/TLS
Validation, authentication and authorization
Encryption
Backups, retention, and geo-redundant storage
Secure cloud environments
Least privilege principle
Compliance requirements for GDPR, HIPAA, etc.
Enable continuous improvement
No AI solution is a one-and-done thing. In fact, good AI evolves and gets better with time. So, set up the protocols and processes for continuous improvement.
Get subject matter experts to perform random tests at regular intervals
Monitor continuously to gauge the performance of your knowledge base
Collect regular feedback on the accuracy, usability and usefulness of the AI knowledge base
Integrate new sources of data as and when they become available
Measure progress against business goals
It’s easy to think of AI knowledge bases as expenses without ROI. Don’t make that mistake. Review its performance on a regular basis. For instance, have the number of queries to the HR team reduced and by how much? Has the knowledge base helped reduce the training budget without affecting employee performance?
Use these metrics to troubleshoot and optimize your AI knowledge base.
For the enterprise team, this might be a lot, given the tech landscape they already manage. An AI knowledge base has a lot of moving parts that need to be balanced delicately. Tune AI’s experts are adept at exactly that.
If you’re looking to implement an AI knowledge base in your enterprise, speak to us today. We’ll show you how you can get it done faster, smarter and better.
Every enterprise’s harsh reality is that they face immense competition every day. There are 520 payment software companies, 466 project management tools, 4012 commercial banks and 33 million small businesses in the US.
Whether you’re making toothpaste or building software, competition is inevitable. As a result, the differentiation in products or features is minimal. Your biggest differentiator - and competitive advantage - is the intangibles, like customer experience, ease of use, personalization, etc.
In short, your success depends not on what you do, but ‘how’ you do things.
But, in an organization of thousands of people, how do you ensure that everyone practices the same culture, embodies the same values, and speaks the same language?
The simple answer is creating common institutional knowledge, where everyone knows what they need to know. That’s easier said than done.
Codifying your how: Traditional learning initiatives
Traditionally, institutional knowledge was imparted through operating manuals, seminars, onboarding lectures, and on-the-job training. The challenge with such outbound knowledge transfer is:
Only the known-knowns are covered
Information given is often generic and not contextual
People forget most of what they learned the moment they step out of the class
And then, the gap between knowing something and applying it to practice is huge. The few examples and workshopping done during training sessions don’t cover every workplace scenario.
For instance, your new employee might learn in training that transparency is a key value in your organization. But what does that mean? Do you share passwords transparently? Do you publish salary data? Do you discuss politics? Do you speak your mind and offer feedback about work?
In response to these challenges, the traditional knowledge base emerged. Originating in the world of computer science — now used widely — a knowledge base is a hierarchical and structured way to store information. A typical knowledge base was like an employee handbook.
The good news is that traditional knowledge bases made information available to the user when they want it. They were indexed and searchable. As the knowledge economy grew, this became common practice.
Yet challenges remain:
Still restricted to known-knowns
Used only structured and documented information
Looking for information was time-consuming
And the employee was entirely alone in their search for knowledge. Unless they asked someone who was kind enough to help, a lot of information was lost in translation. Worse still, for aspects that aren’t deliberately codified, employees saw and learned.
Such unconscious mimicking of behavior has its troubles. Without deliberation and validation, employees might pick up on things that weren’t meant to be, creating counterproductive knowledge.
As author and consultant Ron Ashkenas, writes in the Harvard Business Review, while some institutional knowledge “gets translated into procedures and policies, most of it resides in the heads, hands, and hearts of individual managers and functional experts." Over the years, this knowledge moves away, degrades, or entirely disappears in the natural course of business.
A giant leap forward with an AI knowledge base
Obviously, AI isn’t the bandaid to every problem. However, knowledge management is something AI can meaningfully help with. Here’s how.
Why do you need an AI knowledge base?
A traditional knowledge base is like a book you read to learn something. You can highlight, bookmark, add notes, and carry it in your bag, but that’s that. If you want to find something, you need to remember where, what, and how it was.
An AI knowledge base is like that wise senior employee who has the answers to all questions. They help solve problems and get things done (not just flash information). Often, they might even present answers you didn’t know to ask questions about.
In the real world, the differences are telling.
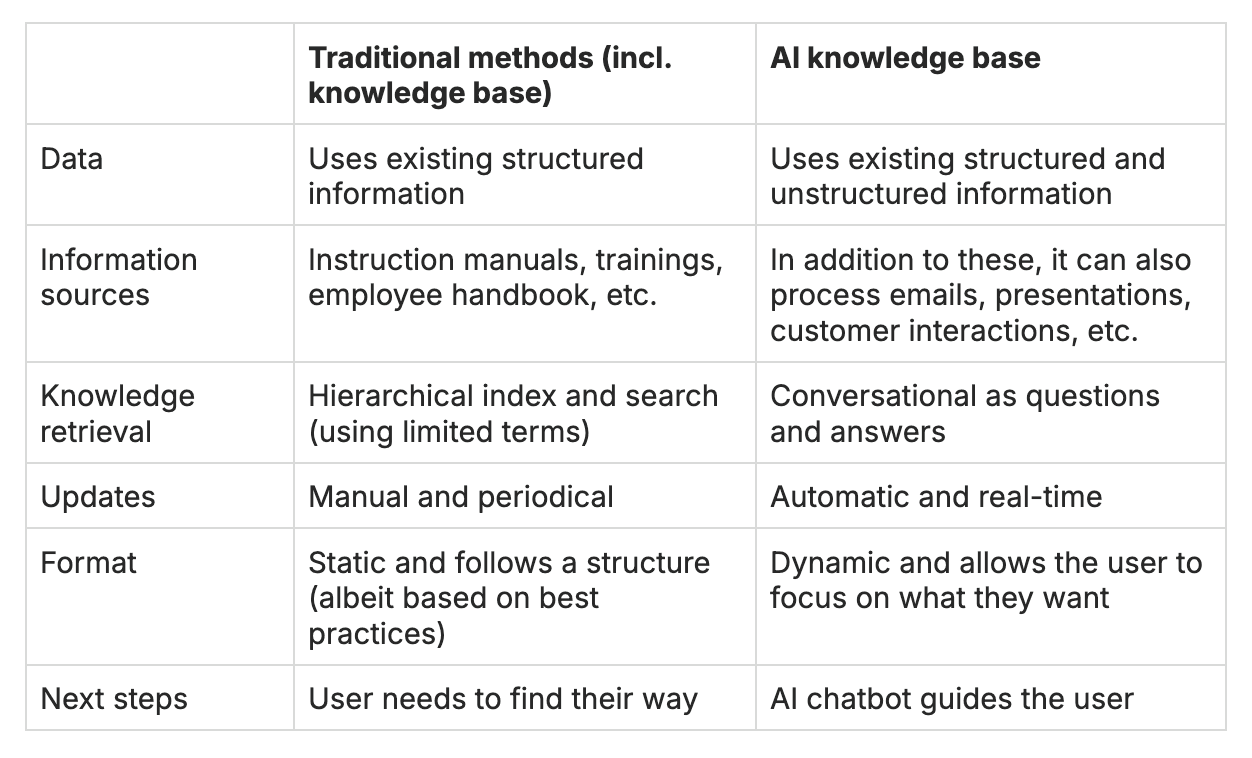
Who is an AI knowledge base for?
The users for an AI knowledge base can be anyone within and outside the organization, depending on your goals and needs. Let’s see some examples.
Customer: Interactions about product information, returns policies, pricing, subscriptions, discounts, etc.
Employee: Understanding company culture, behavior, policies, processes, etc. Imagine the reduction in HR workload if no one ever asked them any questions!
Vendors: Questions about contract renewal, payment timelines, updates, etc. Free up the procurement team of telling a 100 vendors each time that you pay on the first Thursday of every month.
Teams: Standups, updates, schedules, briefs, etc. For instance, integrating AI into your project management system can automate standup notes, understand each other’s work progress, etc. without intrusive conversations or huddles.
Organization: Random bits of information will continue to strengthen institutional knowledge, compounding over time.
How to create an AI knowledge base?
Enterprises already have comprehensive, structured knowledge. You most likely do, too. The best approach is to build on that. Here’s a strategy we’ve found success in implementing.
Define your goals
Ask yourself:
Who is it for? Existing employees, new employees, just the finance team, etc.
What should it do? Reduce emails to HR, save training budget, etc.
What questions should it answer? Boundaries of the knowledge this AI application will possess (critical for security, privacy and compliance)
Centralize your data
When you know the scope of your knowledge base, collect all the data you need for it. We’ve found that a majority of the information is in the following places:
HR platforms: The HRMS, payroll, performance management tools, etc., typically have data around the employee experience.
CX platforms: Customer support tools will have a comprehensive record of customer interactions across channels, including support history, resolutions offered, CSAT/NPS, etc.
Enterprise platforms: ERP, CRM, vendor management, compliance management, finance, etc.
Project management: Whether it’s custom tools like Jira or Trello or the good old Spreadsheet, project management (and internal communication) tools are a goldmine of information about ‘how’ you do things.
Documentation: Past proposals, pitches, presentations, contracts, etc. are stored in SharePoint, OneDrive, or Google Drive. Some of these might be text files, and others might be images, PDFs, etc.
Here’s how we built an agentic assistant for the sales team, leveraging knowledge from 10 years of proposals for a UAE-based event management company.
While doing this, think about access control and data ownership. Define who owns the data you’re integrating and who can access what parts of it. For instance, you might integrate the CRM, while not including any personally identifiable information to your AI solution.
Implement an agentic solution
You can choose something off-the-shelf and integrate all your data into it. However, over the long term, it helps to have a custom solution designed for you and implemented on your infrastructure.
User interface: Build the admin panel and the agentic assistant to enable your teams to use the AI knowledge base.
Design the AI solution: Set up the core servers, retrieval-augmented generation (RAG), feedback storage, database, etc. Choose the LLM and fine-tune it as needed.
Data sources: Integrate the relevant data sources and voila!
Set up governance
With great AI comes great compliance, security and governance risks. Make sure you have comprehensive preventative measures for your AI knowledge base. Some things to think about are:
Data access protocols
SSL/TLS
Validation, authentication and authorization
Encryption
Backups, retention, and geo-redundant storage
Secure cloud environments
Least privilege principle
Compliance requirements for GDPR, HIPAA, etc.
Enable continuous improvement
No AI solution is a one-and-done thing. In fact, good AI evolves and gets better with time. So, set up the protocols and processes for continuous improvement.
Get subject matter experts to perform random tests at regular intervals
Monitor continuously to gauge the performance of your knowledge base
Collect regular feedback on the accuracy, usability and usefulness of the AI knowledge base
Integrate new sources of data as and when they become available
Measure progress against business goals
It’s easy to think of AI knowledge bases as expenses without ROI. Don’t make that mistake. Review its performance on a regular basis. For instance, have the number of queries to the HR team reduced and by how much? Has the knowledge base helped reduce the training budget without affecting employee performance?
Use these metrics to troubleshoot and optimize your AI knowledge base.
For the enterprise team, this might be a lot, given the tech landscape they already manage. An AI knowledge base has a lot of moving parts that need to be balanced delicately. Tune AI’s experts are adept at exactly that.
If you’re looking to implement an AI knowledge base in your enterprise, speak to us today. We’ll show you how you can get it done faster, smarter and better.
Written by
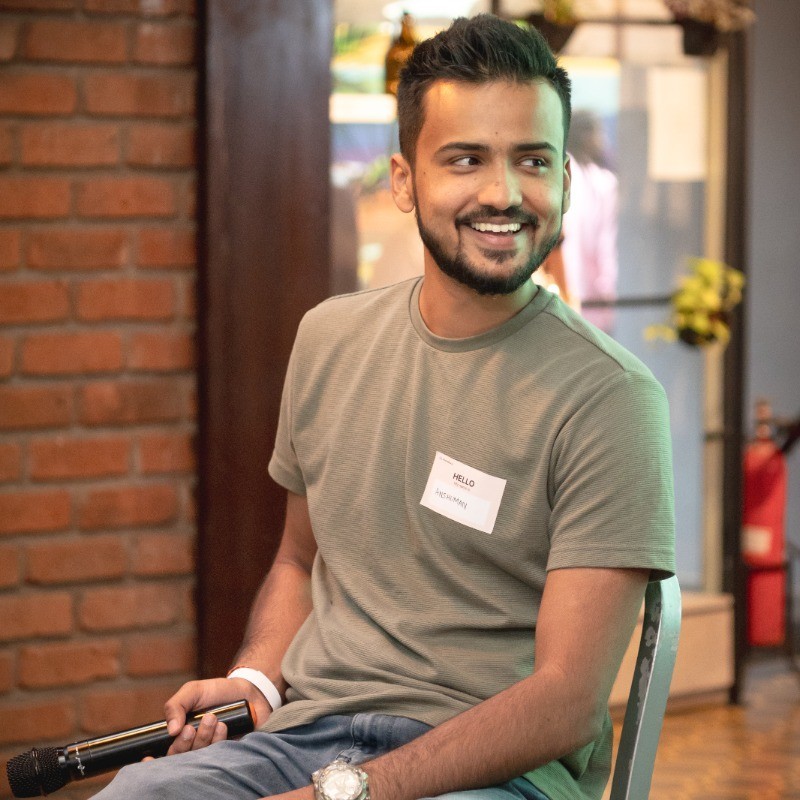
Anshuman Pandey
Co-founder and CEO