Enterprise
AWS Bedrock vs AWS Sagemaker: Same Service, New Hype?
Jul 17, 2024
5 min read
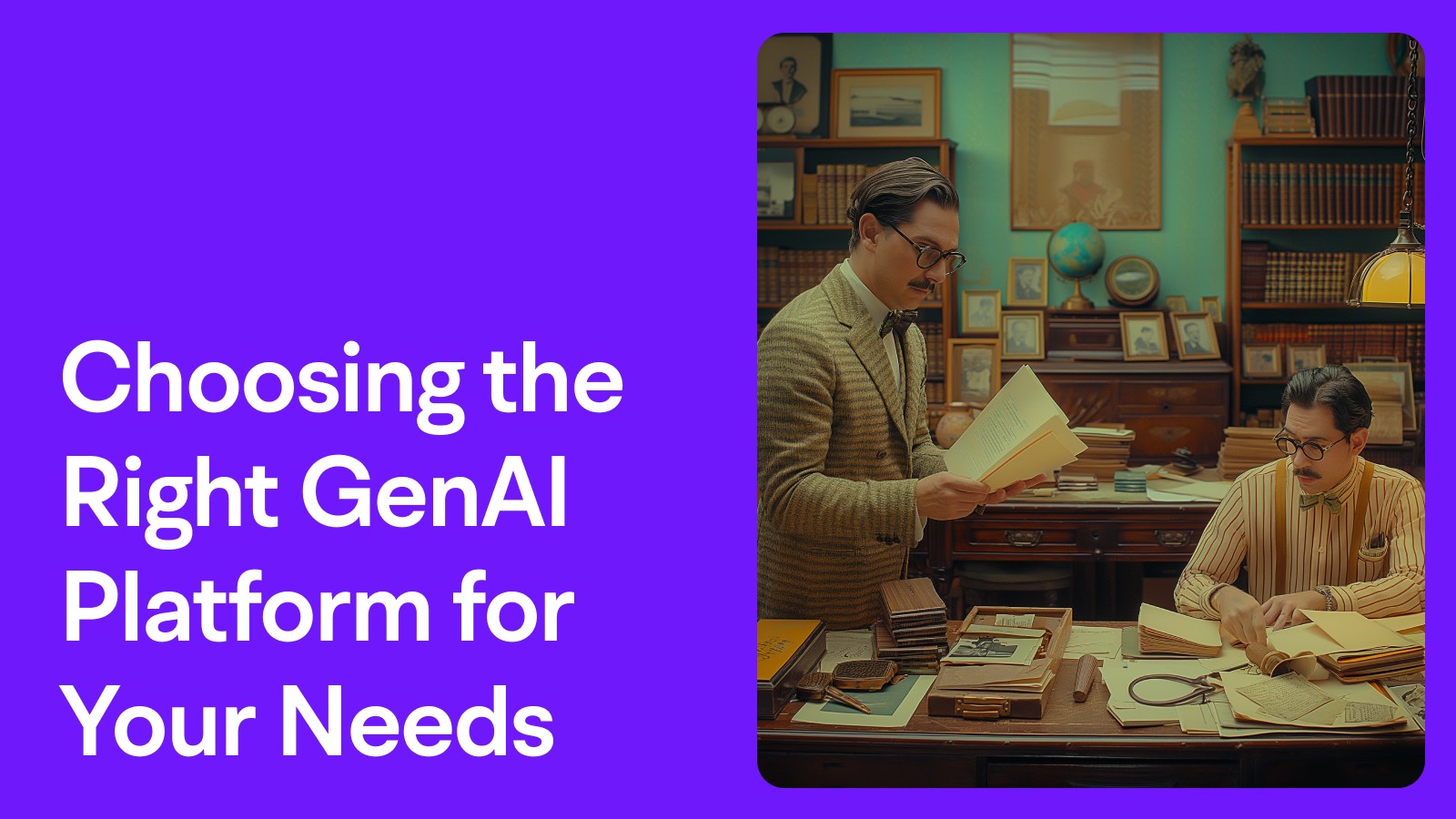
In Today’s rapidly growing AI market, we have quickly exhausted our previous training and deployment pipelines due to the sheer requirements modern Generative AI Models present. This need has paved the way for robust, scalable, and user-friendly tools to develop GenAI models that are not as complicated as classic legends like AWS Sagemaker.
One such service that has had a rather soft launch among developers and has mostly gone unnoticed among the masses is Amazon’s very own GenAI Platform, AWS Bedrock. In this blog post, we explore the various pros and cons of Sagemaker and Bedrock, which should ideally help you decide the service of your choice. So let us go deeper and decide what service should be the catalyst for your upcoming AI Agent!
AWS Bedrock
Amazon Bedrock is a fully managed service hosted by AWS to simplify the integration and use of GenAI models across various projects with simple clicks. The service comes packed with a selection of top-gen AI and Foundational Models with the added benefit of deploying them in a single API without getting into the technical jargon.
A boon for developers worldwide, especially those reliant on and familiar with the promises of AWS, the suite becomes sweeter with the ability to build secure, private, and responsible AI Agents and Applications, without managing or learning how to deal with the underlying infrastructure.
AWS Sagemaker
Moving onto the bigger and more complex service provided by AWS, Sagemaker is a broader Comprehensive Machine Learning Service, which includes utilities and services to adorn most sub-branches of ML from Computer Vision, NLP, and other supervised and unsupervised methods with tools to Build, Train, Deploy and Scale such projects.
Often regarded as one of the most difficult challenges for a fellow ML Enthusiast to conquer, AWS Sagemaker provides a web-based IDE, that unifies all interfaces available on Sagemaker, to cover topics such as Data Preparation, Model Training, Tuning, Deployment and Monitoring.
Technical Comparison
As we try to see a comprehensive difference between the two, let us first differentiate the two services purely on their reliability in performing GenAI tasks:
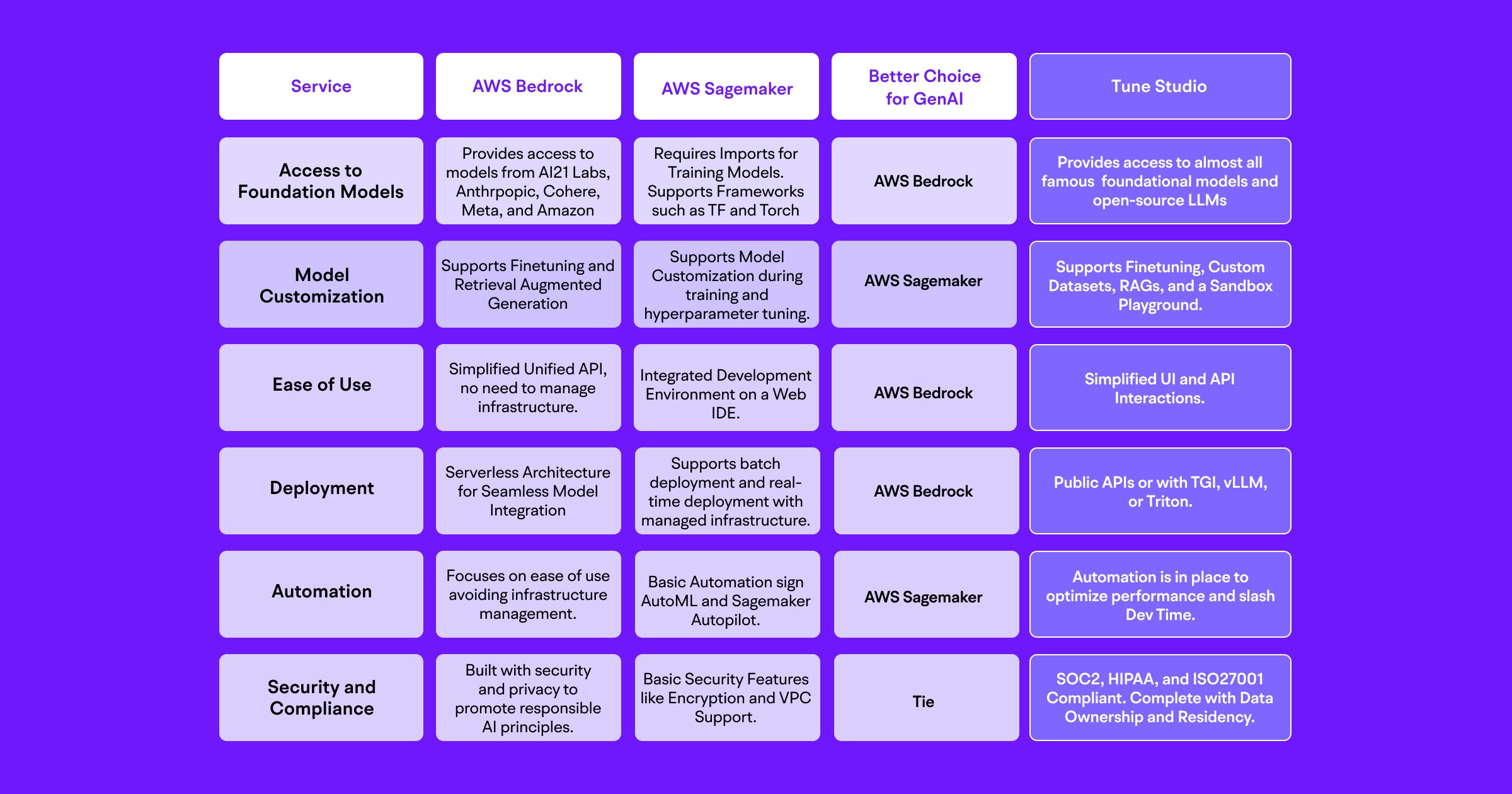
However, do not take our word for the comparisons. Let us see what developers have to say on G2 (Head over to Tune Studio’s G2 to check out what developers have to say about our product!) while comparing both services as you follow this link. One clear distinction that can be drawn between the two can be seen in the demographics for both services.
Let us now come to the question both developers and enterprises are stuck on, where do both of them stand financially?
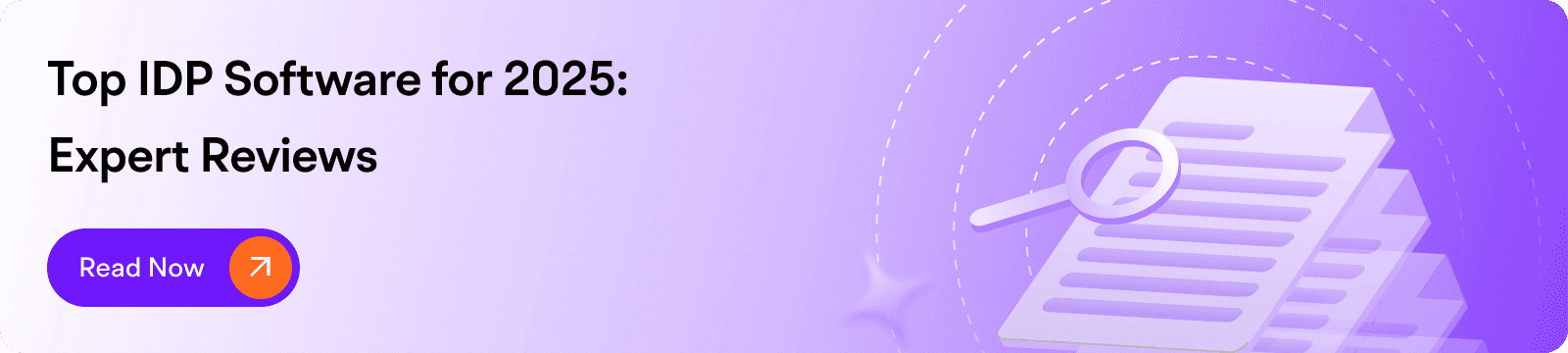
Financial Comparison
As far as financial comparisons are concerned, barring the ease of use aspect proposed by either service, AWS Sagemaker provides a more thorough selection of pricing. By default, both services work on a pay-as-you-use basis, which brings us to an estimation where we are looking for the following conditions to be fulfilled.
Model Deployed: LLaMA 3 8B
Total Inferences: 1 Million
Machine: V100
AWS Sagemaker
When using Sagemaker for hosting a GenAI model, we will have to pay separately for a bunch of microservices, which include the charges for the Hosting, Inference on the Host Server, Storage and lastly the Data Transfer costs incurred while running the inferences and returning and accepting tokens.
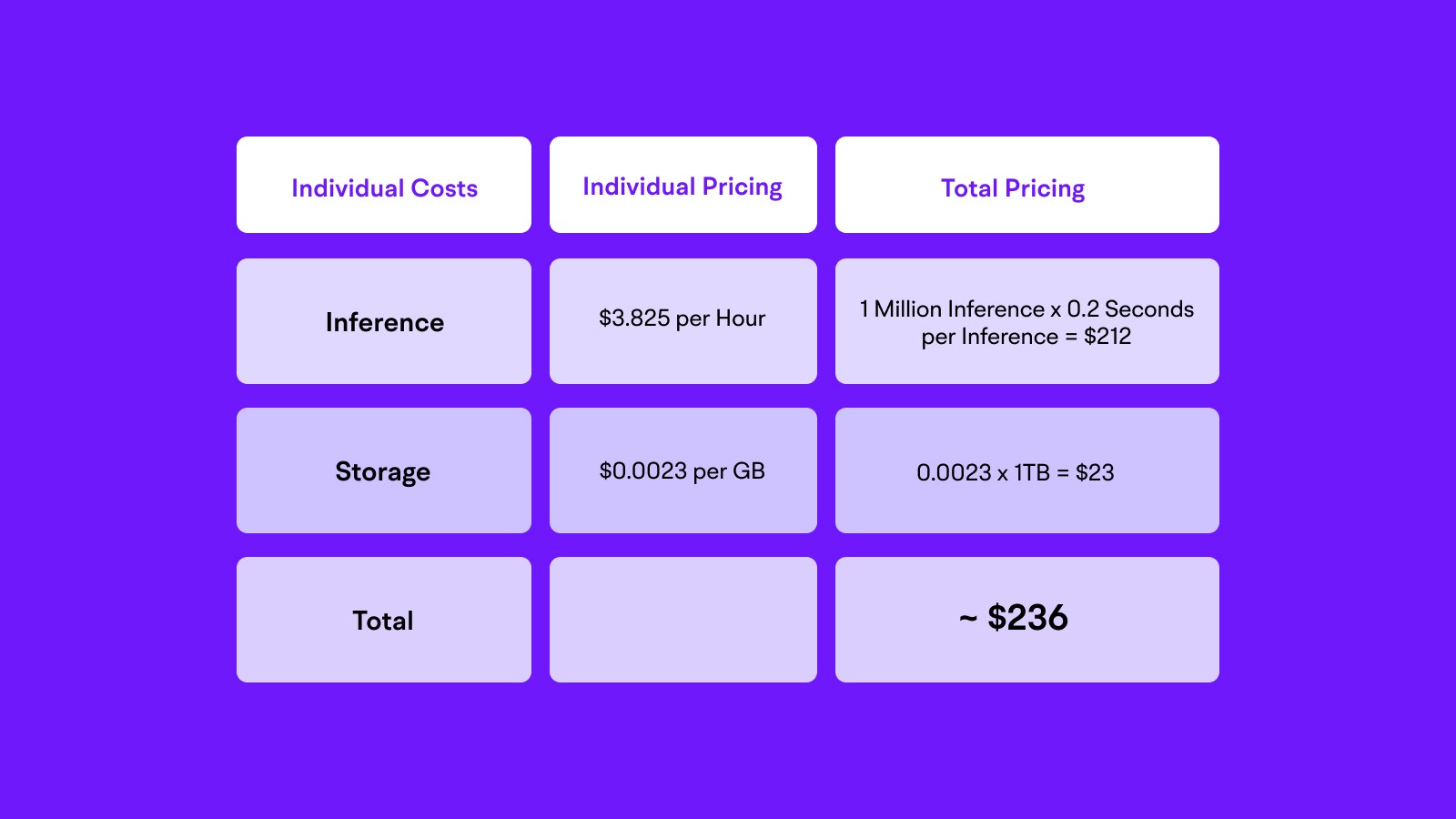
DISCLAIMER: These are just estimates based on potential technical requirements, for more details please refer to the official AWS Pricing Page.
AWS Bedrock
Moving over to Bedrock, the pricing becomes much simpler with the emphasis just on the choice of the foundational model and the amount of Input and Output tokens you are requesting from the same. For the following calculations, we estimate that Input tokens and Output Tokens for each inference will be 50 and 30 respectively. This number of tokens gives us a total of 50 Million (Input) + 30 Million (Output) tokens.
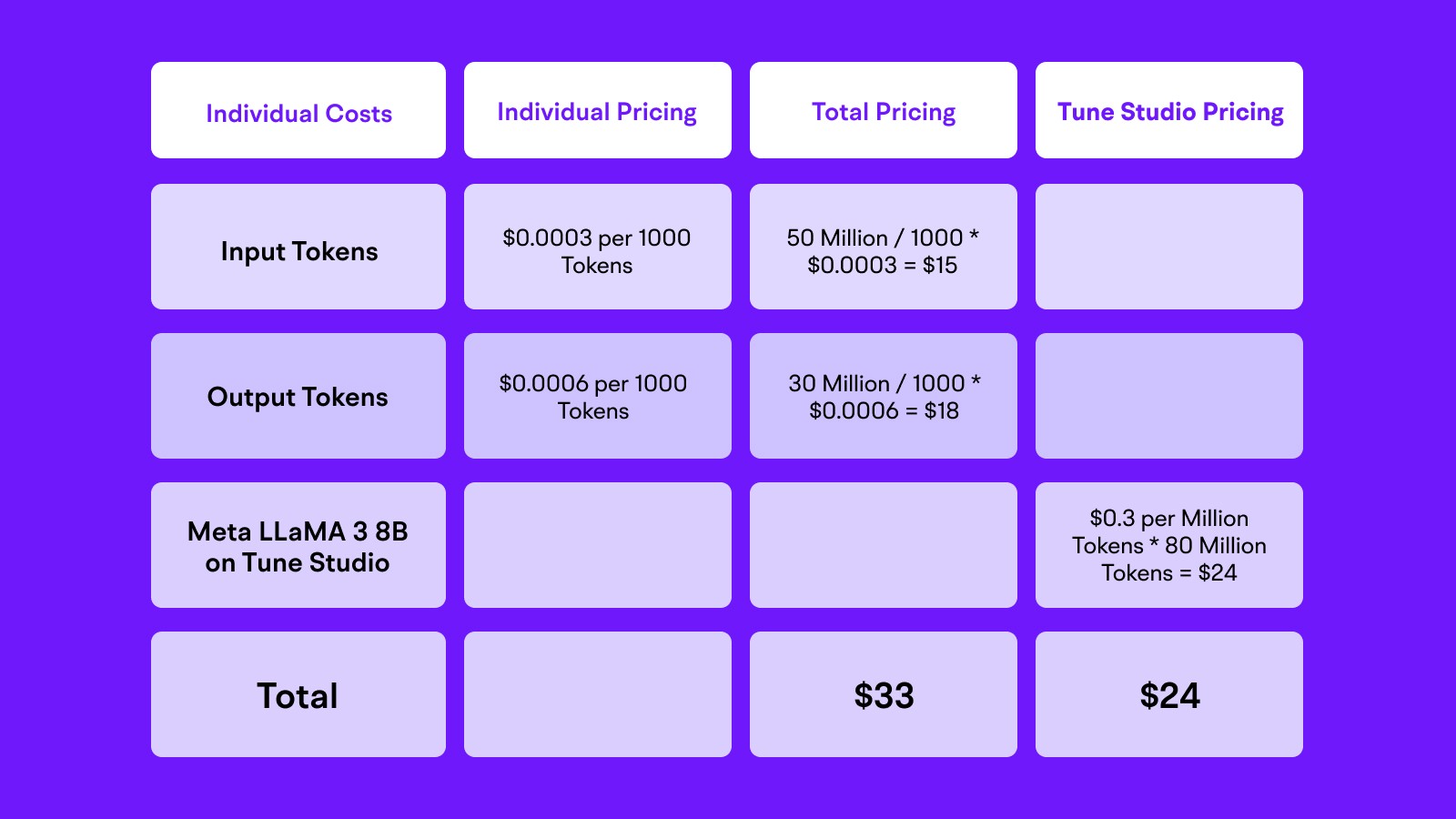
DISCLAIMER: These are just estimates based on potential technical requirements, for more details please refer to the official AWS Pricing Page.
The Better Service?
Now that we have seen the technical and financial limitations of both services, the question remains, which is the best service for your use? We can try to categorize and come to the best decision by checking the following points:
Service Model Architecture: Bedrock’s service model is optimized for inference, while Sagemaker offers unparalleled broader ML Capabilities.
Pricing Structure: Bedrock’s token-based pricing model, which looks effective for inference-heavy tasks, is countered by Sagemaker’s instance-based pricing model which gives you control over your entire pipeline.
Optimization for Inference: Bedrock is optimized for Inference tasks, leading to better costs, but Sagemaker’s instance-based pricing helps you allocate required resources and perform seamless scalable tasks.
Each service is designed for a specific user, and the choice can be made depending on the things you are willing to let go of by using the predefined API calls or managing the entire instance in Sagemaker. The choice includes the balance between cost, control, and the breadth of features both services offer.
However, if you are looking for the best of both worlds, and wanna have the freedom to control your pipeline, but still have the ease of APIs and easy-to-learn LLM Pipeline Management, head over to Tune Studio’s Service page, or get in touch with our team to get the best pricing for your need!
Conclusion
In the comparison, we have discovered the limitations vs pricing balance of both the services offered, which makes Bedrock an ideal choice for casual GenAI developers, as they don’t have to think about surrounding operations involved in GenAI inferences, whereas Sagemaker becomes a better choice for Enterprises due to its modularity with existing systems.
While both present the ability to run inferences seamlessly the difference between the learning curve and pricing seems absurdly huge, which begs the need for a better middle-tier service, which offers easy-to-use technical systems but the ease of APIs, for which you should head to Tune Studio today!
Additional Resources
In Today’s rapidly growing AI market, we have quickly exhausted our previous training and deployment pipelines due to the sheer requirements modern Generative AI Models present. This need has paved the way for robust, scalable, and user-friendly tools to develop GenAI models that are not as complicated as classic legends like AWS Sagemaker.
One such service that has had a rather soft launch among developers and has mostly gone unnoticed among the masses is Amazon’s very own GenAI Platform, AWS Bedrock. In this blog post, we explore the various pros and cons of Sagemaker and Bedrock, which should ideally help you decide the service of your choice. So let us go deeper and decide what service should be the catalyst for your upcoming AI Agent!
AWS Bedrock
Amazon Bedrock is a fully managed service hosted by AWS to simplify the integration and use of GenAI models across various projects with simple clicks. The service comes packed with a selection of top-gen AI and Foundational Models with the added benefit of deploying them in a single API without getting into the technical jargon.
A boon for developers worldwide, especially those reliant on and familiar with the promises of AWS, the suite becomes sweeter with the ability to build secure, private, and responsible AI Agents and Applications, without managing or learning how to deal with the underlying infrastructure.
AWS Sagemaker
Moving onto the bigger and more complex service provided by AWS, Sagemaker is a broader Comprehensive Machine Learning Service, which includes utilities and services to adorn most sub-branches of ML from Computer Vision, NLP, and other supervised and unsupervised methods with tools to Build, Train, Deploy and Scale such projects.
Often regarded as one of the most difficult challenges for a fellow ML Enthusiast to conquer, AWS Sagemaker provides a web-based IDE, that unifies all interfaces available on Sagemaker, to cover topics such as Data Preparation, Model Training, Tuning, Deployment and Monitoring.
Technical Comparison
As we try to see a comprehensive difference between the two, let us first differentiate the two services purely on their reliability in performing GenAI tasks:
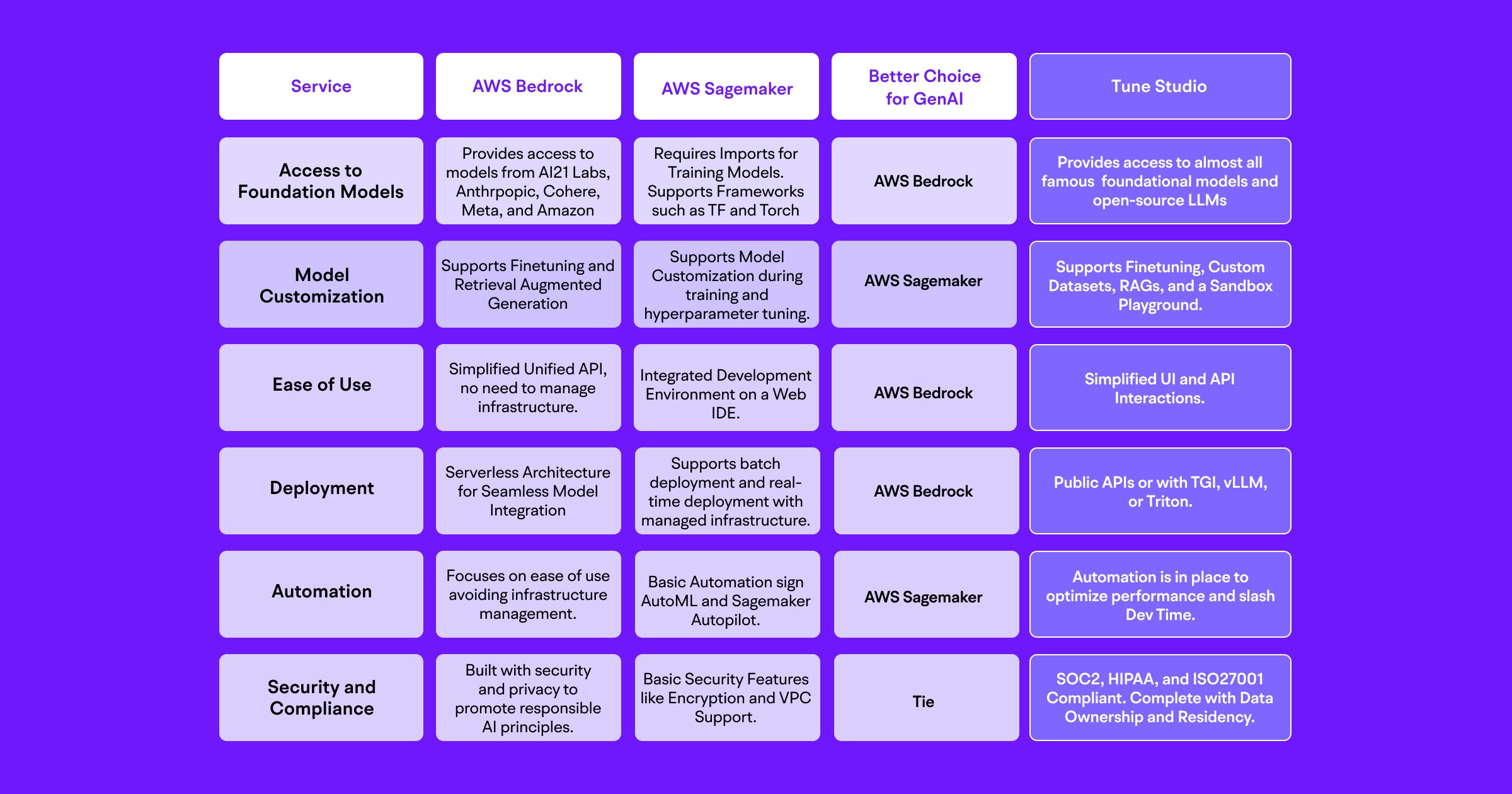
However, do not take our word for the comparisons. Let us see what developers have to say on G2 (Head over to Tune Studio’s G2 to check out what developers have to say about our product!) while comparing both services as you follow this link. One clear distinction that can be drawn between the two can be seen in the demographics for both services.
Let us now come to the question both developers and enterprises are stuck on, where do both of them stand financially?
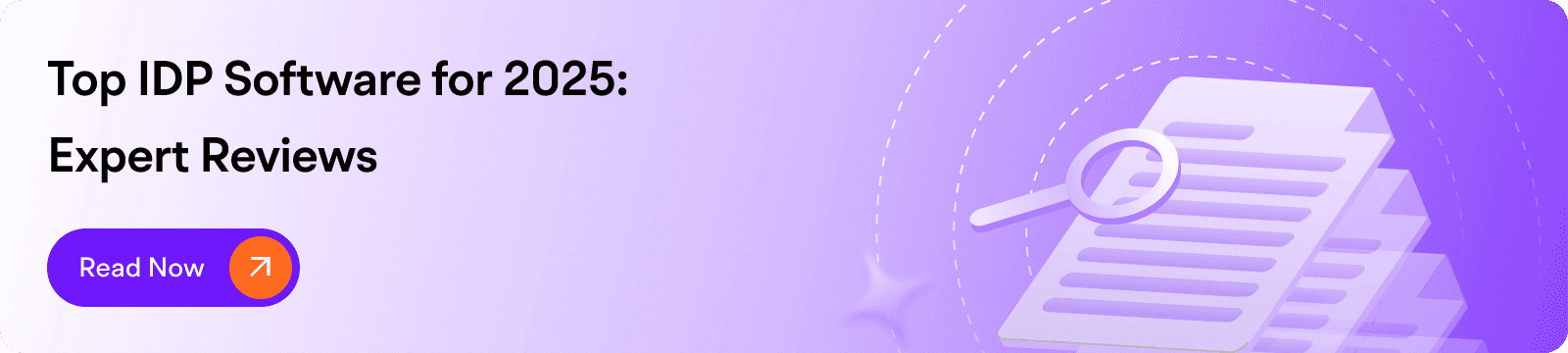
Financial Comparison
As far as financial comparisons are concerned, barring the ease of use aspect proposed by either service, AWS Sagemaker provides a more thorough selection of pricing. By default, both services work on a pay-as-you-use basis, which brings us to an estimation where we are looking for the following conditions to be fulfilled.
Model Deployed: LLaMA 3 8B
Total Inferences: 1 Million
Machine: V100
AWS Sagemaker
When using Sagemaker for hosting a GenAI model, we will have to pay separately for a bunch of microservices, which include the charges for the Hosting, Inference on the Host Server, Storage and lastly the Data Transfer costs incurred while running the inferences and returning and accepting tokens.
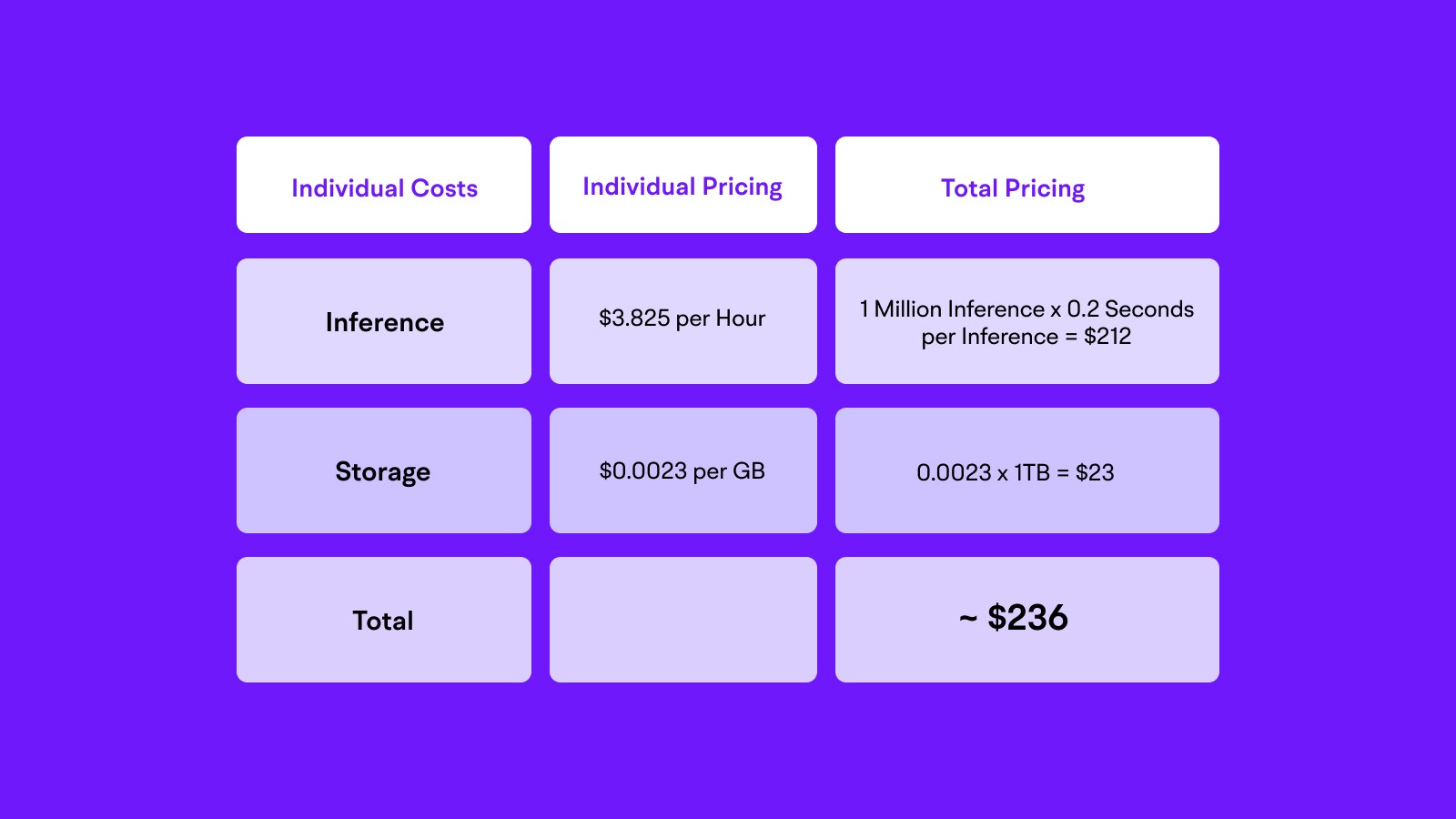
DISCLAIMER: These are just estimates based on potential technical requirements, for more details please refer to the official AWS Pricing Page.
AWS Bedrock
Moving over to Bedrock, the pricing becomes much simpler with the emphasis just on the choice of the foundational model and the amount of Input and Output tokens you are requesting from the same. For the following calculations, we estimate that Input tokens and Output Tokens for each inference will be 50 and 30 respectively. This number of tokens gives us a total of 50 Million (Input) + 30 Million (Output) tokens.
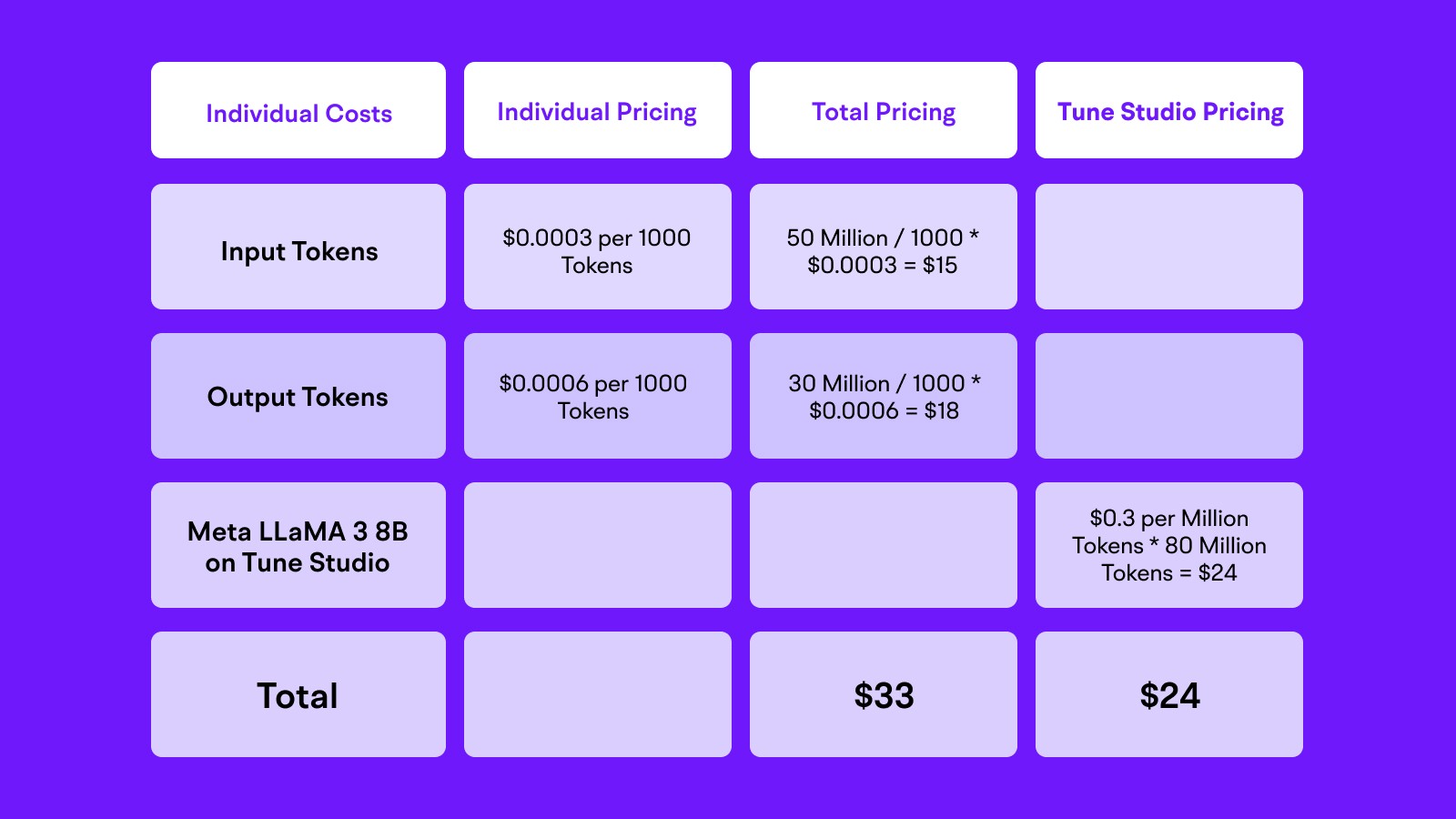
DISCLAIMER: These are just estimates based on potential technical requirements, for more details please refer to the official AWS Pricing Page.
The Better Service?
Now that we have seen the technical and financial limitations of both services, the question remains, which is the best service for your use? We can try to categorize and come to the best decision by checking the following points:
Service Model Architecture: Bedrock’s service model is optimized for inference, while Sagemaker offers unparalleled broader ML Capabilities.
Pricing Structure: Bedrock’s token-based pricing model, which looks effective for inference-heavy tasks, is countered by Sagemaker’s instance-based pricing model which gives you control over your entire pipeline.
Optimization for Inference: Bedrock is optimized for Inference tasks, leading to better costs, but Sagemaker’s instance-based pricing helps you allocate required resources and perform seamless scalable tasks.
Each service is designed for a specific user, and the choice can be made depending on the things you are willing to let go of by using the predefined API calls or managing the entire instance in Sagemaker. The choice includes the balance between cost, control, and the breadth of features both services offer.
However, if you are looking for the best of both worlds, and wanna have the freedom to control your pipeline, but still have the ease of APIs and easy-to-learn LLM Pipeline Management, head over to Tune Studio’s Service page, or get in touch with our team to get the best pricing for your need!
Conclusion
In the comparison, we have discovered the limitations vs pricing balance of both the services offered, which makes Bedrock an ideal choice for casual GenAI developers, as they don’t have to think about surrounding operations involved in GenAI inferences, whereas Sagemaker becomes a better choice for Enterprises due to its modularity with existing systems.
While both present the ability to run inferences seamlessly the difference between the learning curve and pricing seems absurdly huge, which begs the need for a better middle-tier service, which offers easy-to-use technical systems but the ease of APIs, for which you should head to Tune Studio today!
Additional Resources
In Today’s rapidly growing AI market, we have quickly exhausted our previous training and deployment pipelines due to the sheer requirements modern Generative AI Models present. This need has paved the way for robust, scalable, and user-friendly tools to develop GenAI models that are not as complicated as classic legends like AWS Sagemaker.
One such service that has had a rather soft launch among developers and has mostly gone unnoticed among the masses is Amazon’s very own GenAI Platform, AWS Bedrock. In this blog post, we explore the various pros and cons of Sagemaker and Bedrock, which should ideally help you decide the service of your choice. So let us go deeper and decide what service should be the catalyst for your upcoming AI Agent!
AWS Bedrock
Amazon Bedrock is a fully managed service hosted by AWS to simplify the integration and use of GenAI models across various projects with simple clicks. The service comes packed with a selection of top-gen AI and Foundational Models with the added benefit of deploying them in a single API without getting into the technical jargon.
A boon for developers worldwide, especially those reliant on and familiar with the promises of AWS, the suite becomes sweeter with the ability to build secure, private, and responsible AI Agents and Applications, without managing or learning how to deal with the underlying infrastructure.
AWS Sagemaker
Moving onto the bigger and more complex service provided by AWS, Sagemaker is a broader Comprehensive Machine Learning Service, which includes utilities and services to adorn most sub-branches of ML from Computer Vision, NLP, and other supervised and unsupervised methods with tools to Build, Train, Deploy and Scale such projects.
Often regarded as one of the most difficult challenges for a fellow ML Enthusiast to conquer, AWS Sagemaker provides a web-based IDE, that unifies all interfaces available on Sagemaker, to cover topics such as Data Preparation, Model Training, Tuning, Deployment and Monitoring.
Technical Comparison
As we try to see a comprehensive difference between the two, let us first differentiate the two services purely on their reliability in performing GenAI tasks:
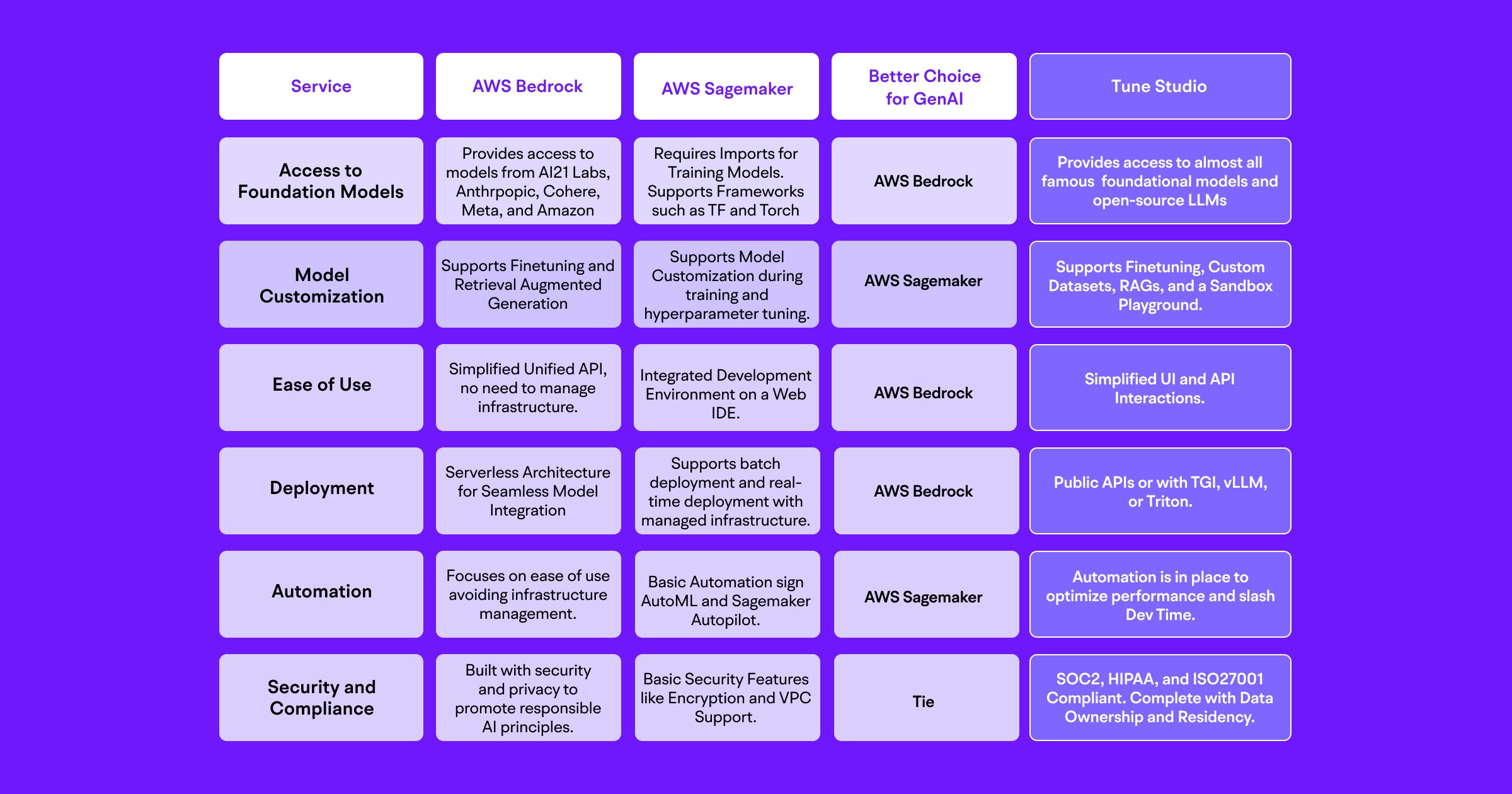
However, do not take our word for the comparisons. Let us see what developers have to say on G2 (Head over to Tune Studio’s G2 to check out what developers have to say about our product!) while comparing both services as you follow this link. One clear distinction that can be drawn between the two can be seen in the demographics for both services.
Let us now come to the question both developers and enterprises are stuck on, where do both of them stand financially?
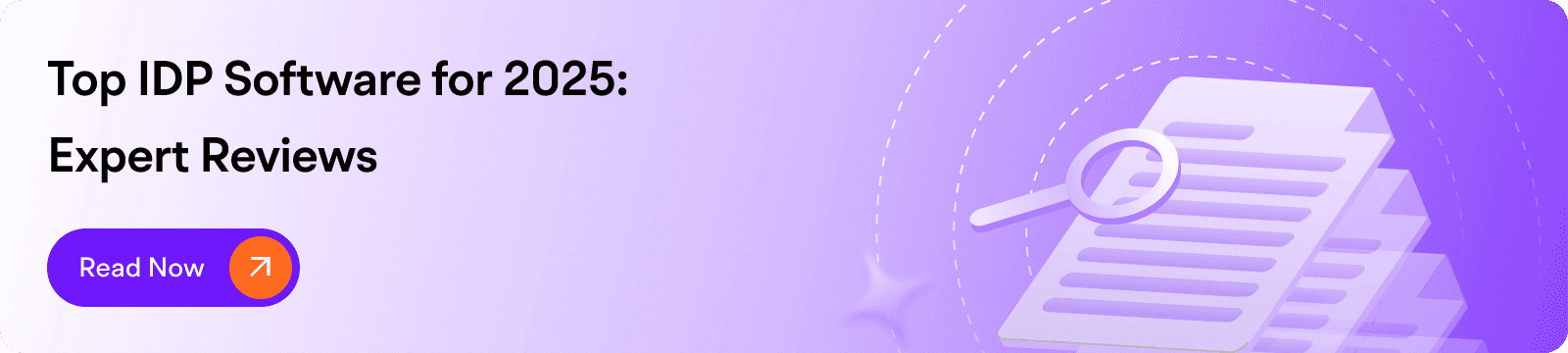
Financial Comparison
As far as financial comparisons are concerned, barring the ease of use aspect proposed by either service, AWS Sagemaker provides a more thorough selection of pricing. By default, both services work on a pay-as-you-use basis, which brings us to an estimation where we are looking for the following conditions to be fulfilled.
Model Deployed: LLaMA 3 8B
Total Inferences: 1 Million
Machine: V100
AWS Sagemaker
When using Sagemaker for hosting a GenAI model, we will have to pay separately for a bunch of microservices, which include the charges for the Hosting, Inference on the Host Server, Storage and lastly the Data Transfer costs incurred while running the inferences and returning and accepting tokens.
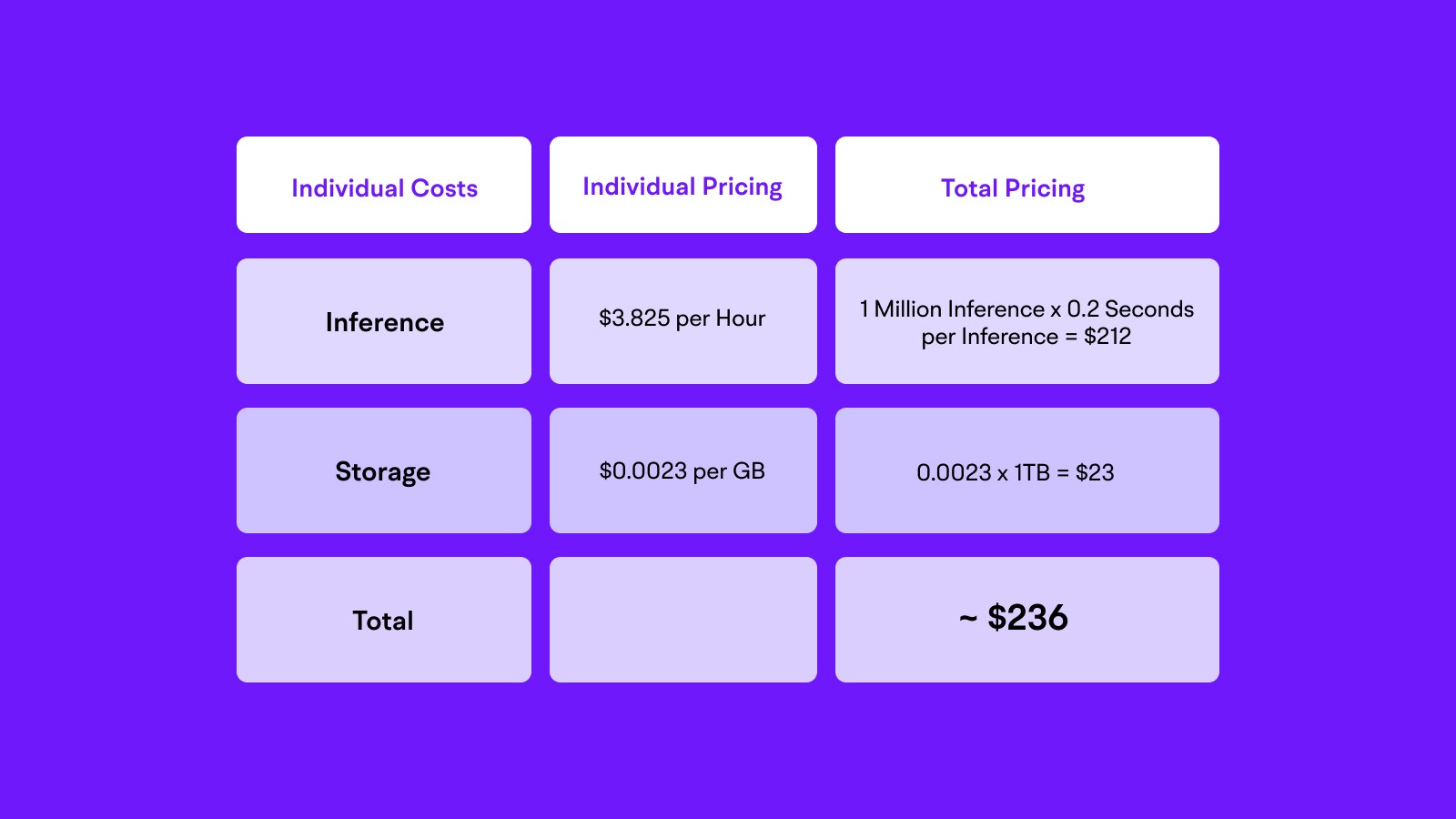
DISCLAIMER: These are just estimates based on potential technical requirements, for more details please refer to the official AWS Pricing Page.
AWS Bedrock
Moving over to Bedrock, the pricing becomes much simpler with the emphasis just on the choice of the foundational model and the amount of Input and Output tokens you are requesting from the same. For the following calculations, we estimate that Input tokens and Output Tokens for each inference will be 50 and 30 respectively. This number of tokens gives us a total of 50 Million (Input) + 30 Million (Output) tokens.
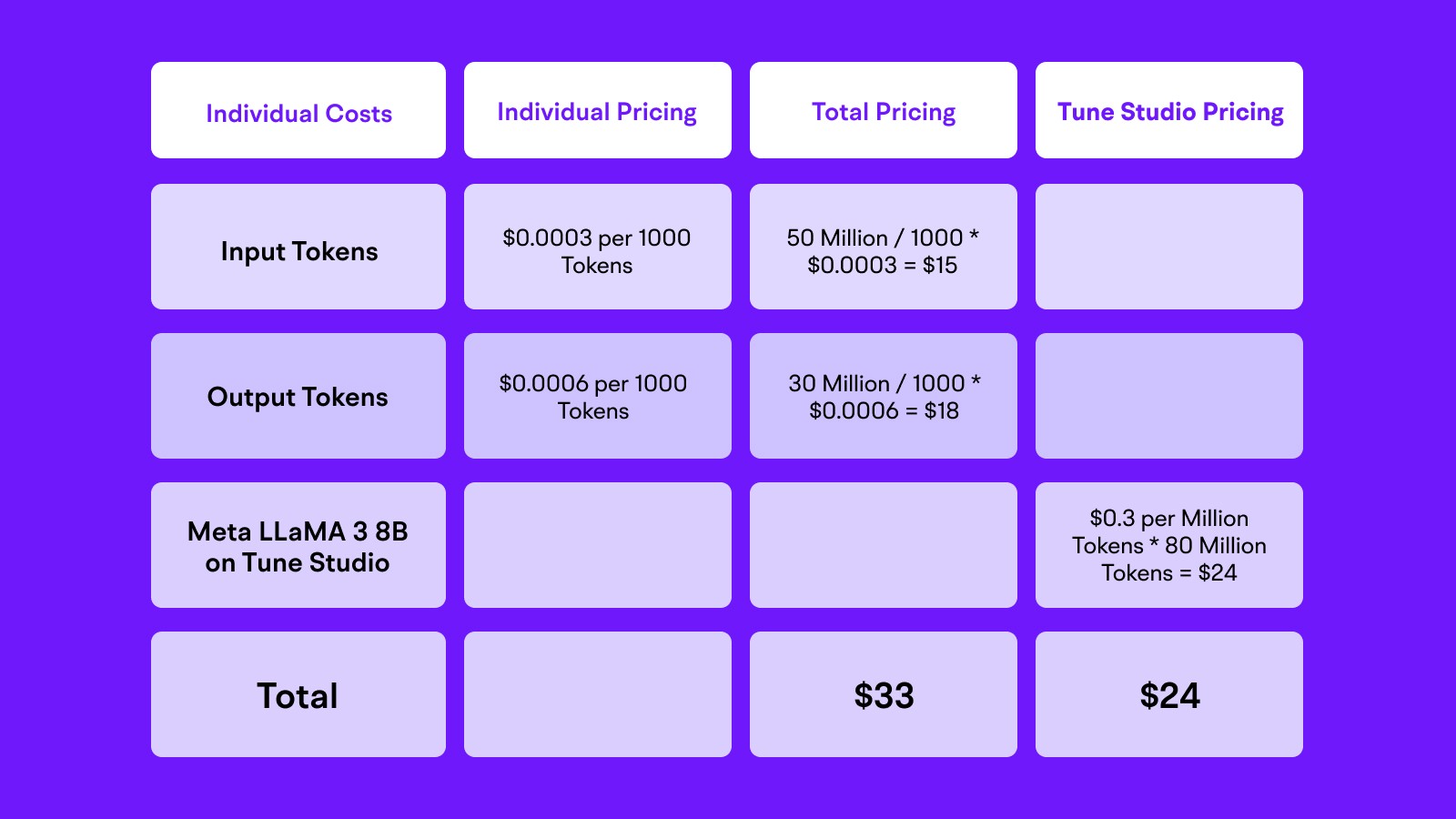
DISCLAIMER: These are just estimates based on potential technical requirements, for more details please refer to the official AWS Pricing Page.
The Better Service?
Now that we have seen the technical and financial limitations of both services, the question remains, which is the best service for your use? We can try to categorize and come to the best decision by checking the following points:
Service Model Architecture: Bedrock’s service model is optimized for inference, while Sagemaker offers unparalleled broader ML Capabilities.
Pricing Structure: Bedrock’s token-based pricing model, which looks effective for inference-heavy tasks, is countered by Sagemaker’s instance-based pricing model which gives you control over your entire pipeline.
Optimization for Inference: Bedrock is optimized for Inference tasks, leading to better costs, but Sagemaker’s instance-based pricing helps you allocate required resources and perform seamless scalable tasks.
Each service is designed for a specific user, and the choice can be made depending on the things you are willing to let go of by using the predefined API calls or managing the entire instance in Sagemaker. The choice includes the balance between cost, control, and the breadth of features both services offer.
However, if you are looking for the best of both worlds, and wanna have the freedom to control your pipeline, but still have the ease of APIs and easy-to-learn LLM Pipeline Management, head over to Tune Studio’s Service page, or get in touch with our team to get the best pricing for your need!
Conclusion
In the comparison, we have discovered the limitations vs pricing balance of both the services offered, which makes Bedrock an ideal choice for casual GenAI developers, as they don’t have to think about surrounding operations involved in GenAI inferences, whereas Sagemaker becomes a better choice for Enterprises due to its modularity with existing systems.
While both present the ability to run inferences seamlessly the difference between the learning curve and pricing seems absurdly huge, which begs the need for a better middle-tier service, which offers easy-to-use technical systems but the ease of APIs, for which you should head to Tune Studio today!
Additional Resources
Written by
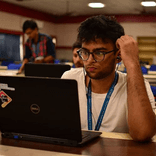
Aryan Kargwal
Data Evangelist
Edited by

Abhishek Mishra
DevRel Engineer