Enterprise
Keeping Your Data Safe: The Security Advantages of On-Premise AI
Oct 22, 2024
5 min read

Over the last fifteen years, cloud infrastructure gained favor primarily due to its scalability. While cost was a huge factor, CTOs were soon disillusioned of cloud being cheaper (basically, the total cost of ownership of cloud nearly matched on-prem for most applications).
However, setting up a data center with all its bells and whistles of real estate, electricity, cooling, etc. for enormous AI applications - which are still in experimental stages - is just much. McKinsey’s Mark Gu illustrates this.
We came across a problem a few years ago with a company whose challenge was to monitor a massive volume of market transactions.
The company’s existing infrastructure and software on premises just wasn’t capable of handling trillions of transactions a day. So instead of rebuilding on-premises and traditional techniques, company leaders said, ‘Why don’t we try this cloud thing? It allows us to experiment quickly and then scale almost to infinite size in terms of the amount of analytics we can do. (emphasis ours)
However, as the industry matures, there is one huge learning: The opportunity costs of leaving on-prem have gone up, especially around security.
Cloud security concerns around GenAI applications
If you’re considering AI — especially GenAI — for your organization, irrespective of the use cases, you need to think about the following.
Larger attack surface: If you’re integrating AI into your business workflows, you’re essentially opening up the workflows (and the ERPs) to attack from malicious actors. Besides theft, you also face the threat of data poisoning, where attackers manipulate training data to impact model behavior.
GenAI tool policy: If you’re using a vendor’s proprietary model for your GenAI applications, using their API, how can you be sure that they’re not using your data to train their models? Even if they have policies around that, how can you be sure they’re followed diligently?
Data loss due to user error: As your employees learn to use Generative AI, they may inadvertently expose your data. Imagine something as simple as your employee posting your company financials to ChatGPT with a prompt that says, “ELI5 this report”! You wouldn’t want ChatGPT to know your company's financials, or worse, train their future models with them.
Model theft: Every smart enterprise customizes and fine-tunes the LLMs it uses. This is done with proprietary enterprise data such as customer information, financial data, and other organizational knowledge.
Cloud implementation of such models is prone to model theft. A threat actor might sneak their way to querying your model and reverse engineer a competitive algorithm with its responses.
While addressing all of the security concerns with Generative AI needs a strategic risk-based approach, on-prem implementation must be a critical part of it.
The Security Advantages of On-Premise AI
Simply put, on-premise AI refers to the implementation and deployment of LLMs and chatbots within the boundaries of an organization's physical infrastructure. This offers benefits of security, privacy, compliance, flexibility, and even performance in several cases. Here’s how.
Better data control
When you implement your AI applications on-prem, you have complete control over the data, its storage, and usage.
Access and availability: As data remains within the physical boundaries of your enterprise, risks of data breaches are minimized. This is especially critical if you use sensitive data, like personally identifiable information, medical records, classified documents, etc.
Data minimization and sharing: With on-prem deployments, you reduce the need to share data with third-party cloud providers, ergo better security. Moreover, you can implement data minimization practices to limit your storage to only the necessary data, shrinking the attack surface.
Data protection: In the world of knowledge work, trade secrets are the only competitive advantage. On-prem offers better control and protection of trade secrets from unauthorized access or use. You can even set up network isolation for specific data to completely cordon it off the Internet.
Better regulatory compliance
Laws around data privacy and security are very strict across the globe. If you work in highly regulated industries like financial services, healthcare, pharmaceuticals, etc. you’re more likely to come under the microscope. On-prem implementations offer greater advantages for improving your security posture.
Tailored compliance: On-prem deployments are more flexible to tailor data/network/storage security protocols to meet specific industry standards.
For instance, until 2020, FDA approved only the use of locked algorithms, i.e, once you train your model, you deploy as-is — it can’t continuously learn. Even now, it only authorizes full AI/ML algorithms with predetermined change control plans. On-prem implementations make it easier to handle these requirements from time to time.
Data residency: Some countries have data residency laws which require organizations to store data within its borders. For instance, Australia has restrictions on health data leaving the country. Japan requires data subjects to opt-in for cross-border transfer of data.
Data governance: On-prem deployments offer greater customizability to set data governance policies around access, authorization, authentication, retention, encryption, etc.
Better performance
Typically, cloud is understood to have better performance because it’s scalable. As technology has evolved, today, the opposite can also be true.

NVIDIA DGX Superpod (source: NVIDIA)
Latency: Most GenAI chatbots require low latency. On-prem implementation allows greater customizability, so you can set up your infra to reduce latency and increase response times.
High-performance: For GenAI apps that need high computational resources, you can design suitable hardware and infra to enhance performance. The NVIDIA DGX Systems that we use for on-prem deployments is just as powerful and effective as any of the systems available on cloud.
As you can see, across all fronts, on-prem implementations of Generative AI offer significant security advantages. Moreover, if you’re using open-source models, the control, compliance, and performance benefits multiply.
Sounds too good to be true? Allow us to show you hard facts. Speak to Tune AI experts to get a point-by-point comparison of on-prem and cloud deployments for your applications.
If cloud is better for you, we will tell you as much!
Over the last fifteen years, cloud infrastructure gained favor primarily due to its scalability. While cost was a huge factor, CTOs were soon disillusioned of cloud being cheaper (basically, the total cost of ownership of cloud nearly matched on-prem for most applications).
However, setting up a data center with all its bells and whistles of real estate, electricity, cooling, etc. for enormous AI applications - which are still in experimental stages - is just much. McKinsey’s Mark Gu illustrates this.
We came across a problem a few years ago with a company whose challenge was to monitor a massive volume of market transactions.
The company’s existing infrastructure and software on premises just wasn’t capable of handling trillions of transactions a day. So instead of rebuilding on-premises and traditional techniques, company leaders said, ‘Why don’t we try this cloud thing? It allows us to experiment quickly and then scale almost to infinite size in terms of the amount of analytics we can do. (emphasis ours)
However, as the industry matures, there is one huge learning: The opportunity costs of leaving on-prem have gone up, especially around security.
Cloud security concerns around GenAI applications
If you’re considering AI — especially GenAI — for your organization, irrespective of the use cases, you need to think about the following.
Larger attack surface: If you’re integrating AI into your business workflows, you’re essentially opening up the workflows (and the ERPs) to attack from malicious actors. Besides theft, you also face the threat of data poisoning, where attackers manipulate training data to impact model behavior.
GenAI tool policy: If you’re using a vendor’s proprietary model for your GenAI applications, using their API, how can you be sure that they’re not using your data to train their models? Even if they have policies around that, how can you be sure they’re followed diligently?
Data loss due to user error: As your employees learn to use Generative AI, they may inadvertently expose your data. Imagine something as simple as your employee posting your company financials to ChatGPT with a prompt that says, “ELI5 this report”! You wouldn’t want ChatGPT to know your company's financials, or worse, train their future models with them.
Model theft: Every smart enterprise customizes and fine-tunes the LLMs it uses. This is done with proprietary enterprise data such as customer information, financial data, and other organizational knowledge.
Cloud implementation of such models is prone to model theft. A threat actor might sneak their way to querying your model and reverse engineer a competitive algorithm with its responses.
While addressing all of the security concerns with Generative AI needs a strategic risk-based approach, on-prem implementation must be a critical part of it.
The Security Advantages of On-Premise AI
Simply put, on-premise AI refers to the implementation and deployment of LLMs and chatbots within the boundaries of an organization's physical infrastructure. This offers benefits of security, privacy, compliance, flexibility, and even performance in several cases. Here’s how.
Better data control
When you implement your AI applications on-prem, you have complete control over the data, its storage, and usage.
Access and availability: As data remains within the physical boundaries of your enterprise, risks of data breaches are minimized. This is especially critical if you use sensitive data, like personally identifiable information, medical records, classified documents, etc.
Data minimization and sharing: With on-prem deployments, you reduce the need to share data with third-party cloud providers, ergo better security. Moreover, you can implement data minimization practices to limit your storage to only the necessary data, shrinking the attack surface.
Data protection: In the world of knowledge work, trade secrets are the only competitive advantage. On-prem offers better control and protection of trade secrets from unauthorized access or use. You can even set up network isolation for specific data to completely cordon it off the Internet.
Better regulatory compliance
Laws around data privacy and security are very strict across the globe. If you work in highly regulated industries like financial services, healthcare, pharmaceuticals, etc. you’re more likely to come under the microscope. On-prem implementations offer greater advantages for improving your security posture.
Tailored compliance: On-prem deployments are more flexible to tailor data/network/storage security protocols to meet specific industry standards.
For instance, until 2020, FDA approved only the use of locked algorithms, i.e, once you train your model, you deploy as-is — it can’t continuously learn. Even now, it only authorizes full AI/ML algorithms with predetermined change control plans. On-prem implementations make it easier to handle these requirements from time to time.
Data residency: Some countries have data residency laws which require organizations to store data within its borders. For instance, Australia has restrictions on health data leaving the country. Japan requires data subjects to opt-in for cross-border transfer of data.
Data governance: On-prem deployments offer greater customizability to set data governance policies around access, authorization, authentication, retention, encryption, etc.
Better performance
Typically, cloud is understood to have better performance because it’s scalable. As technology has evolved, today, the opposite can also be true.

NVIDIA DGX Superpod (source: NVIDIA)
Latency: Most GenAI chatbots require low latency. On-prem implementation allows greater customizability, so you can set up your infra to reduce latency and increase response times.
High-performance: For GenAI apps that need high computational resources, you can design suitable hardware and infra to enhance performance. The NVIDIA DGX Systems that we use for on-prem deployments is just as powerful and effective as any of the systems available on cloud.
As you can see, across all fronts, on-prem implementations of Generative AI offer significant security advantages. Moreover, if you’re using open-source models, the control, compliance, and performance benefits multiply.
Sounds too good to be true? Allow us to show you hard facts. Speak to Tune AI experts to get a point-by-point comparison of on-prem and cloud deployments for your applications.
If cloud is better for you, we will tell you as much!
Over the last fifteen years, cloud infrastructure gained favor primarily due to its scalability. While cost was a huge factor, CTOs were soon disillusioned of cloud being cheaper (basically, the total cost of ownership of cloud nearly matched on-prem for most applications).
However, setting up a data center with all its bells and whistles of real estate, electricity, cooling, etc. for enormous AI applications - which are still in experimental stages - is just much. McKinsey’s Mark Gu illustrates this.
We came across a problem a few years ago with a company whose challenge was to monitor a massive volume of market transactions.
The company’s existing infrastructure and software on premises just wasn’t capable of handling trillions of transactions a day. So instead of rebuilding on-premises and traditional techniques, company leaders said, ‘Why don’t we try this cloud thing? It allows us to experiment quickly and then scale almost to infinite size in terms of the amount of analytics we can do. (emphasis ours)
However, as the industry matures, there is one huge learning: The opportunity costs of leaving on-prem have gone up, especially around security.
Cloud security concerns around GenAI applications
If you’re considering AI — especially GenAI — for your organization, irrespective of the use cases, you need to think about the following.
Larger attack surface: If you’re integrating AI into your business workflows, you’re essentially opening up the workflows (and the ERPs) to attack from malicious actors. Besides theft, you also face the threat of data poisoning, where attackers manipulate training data to impact model behavior.
GenAI tool policy: If you’re using a vendor’s proprietary model for your GenAI applications, using their API, how can you be sure that they’re not using your data to train their models? Even if they have policies around that, how can you be sure they’re followed diligently?
Data loss due to user error: As your employees learn to use Generative AI, they may inadvertently expose your data. Imagine something as simple as your employee posting your company financials to ChatGPT with a prompt that says, “ELI5 this report”! You wouldn’t want ChatGPT to know your company's financials, or worse, train their future models with them.
Model theft: Every smart enterprise customizes and fine-tunes the LLMs it uses. This is done with proprietary enterprise data such as customer information, financial data, and other organizational knowledge.
Cloud implementation of such models is prone to model theft. A threat actor might sneak their way to querying your model and reverse engineer a competitive algorithm with its responses.
While addressing all of the security concerns with Generative AI needs a strategic risk-based approach, on-prem implementation must be a critical part of it.
The Security Advantages of On-Premise AI
Simply put, on-premise AI refers to the implementation and deployment of LLMs and chatbots within the boundaries of an organization's physical infrastructure. This offers benefits of security, privacy, compliance, flexibility, and even performance in several cases. Here’s how.
Better data control
When you implement your AI applications on-prem, you have complete control over the data, its storage, and usage.
Access and availability: As data remains within the physical boundaries of your enterprise, risks of data breaches are minimized. This is especially critical if you use sensitive data, like personally identifiable information, medical records, classified documents, etc.
Data minimization and sharing: With on-prem deployments, you reduce the need to share data with third-party cloud providers, ergo better security. Moreover, you can implement data minimization practices to limit your storage to only the necessary data, shrinking the attack surface.
Data protection: In the world of knowledge work, trade secrets are the only competitive advantage. On-prem offers better control and protection of trade secrets from unauthorized access or use. You can even set up network isolation for specific data to completely cordon it off the Internet.
Better regulatory compliance
Laws around data privacy and security are very strict across the globe. If you work in highly regulated industries like financial services, healthcare, pharmaceuticals, etc. you’re more likely to come under the microscope. On-prem implementations offer greater advantages for improving your security posture.
Tailored compliance: On-prem deployments are more flexible to tailor data/network/storage security protocols to meet specific industry standards.
For instance, until 2020, FDA approved only the use of locked algorithms, i.e, once you train your model, you deploy as-is — it can’t continuously learn. Even now, it only authorizes full AI/ML algorithms with predetermined change control plans. On-prem implementations make it easier to handle these requirements from time to time.
Data residency: Some countries have data residency laws which require organizations to store data within its borders. For instance, Australia has restrictions on health data leaving the country. Japan requires data subjects to opt-in for cross-border transfer of data.
Data governance: On-prem deployments offer greater customizability to set data governance policies around access, authorization, authentication, retention, encryption, etc.
Better performance
Typically, cloud is understood to have better performance because it’s scalable. As technology has evolved, today, the opposite can also be true.

NVIDIA DGX Superpod (source: NVIDIA)
Latency: Most GenAI chatbots require low latency. On-prem implementation allows greater customizability, so you can set up your infra to reduce latency and increase response times.
High-performance: For GenAI apps that need high computational resources, you can design suitable hardware and infra to enhance performance. The NVIDIA DGX Systems that we use for on-prem deployments is just as powerful and effective as any of the systems available on cloud.
As you can see, across all fronts, on-prem implementations of Generative AI offer significant security advantages. Moreover, if you’re using open-source models, the control, compliance, and performance benefits multiply.
Sounds too good to be true? Allow us to show you hard facts. Speak to Tune AI experts to get a point-by-point comparison of on-prem and cloud deployments for your applications.
If cloud is better for you, we will tell you as much!
Written by
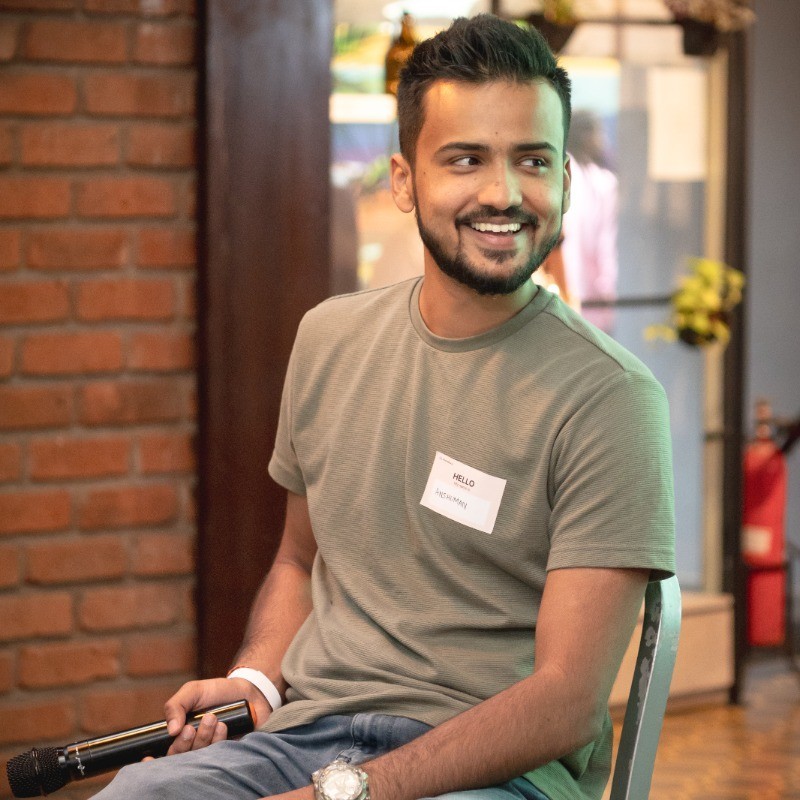
Anshuman Pandey
Co-founder and CEO